ビジネスアプリケーションの概要¶
このセクションでは、各業界において想定される予測モデルの使用例を示すために、さまざまなユースケースの簡単な概要を、 AIアプリとともに提供します。
AIアプリでは、DataRobotでモデルの構築やパフォーマンス評価を行わなくても、ノーコードインターフェイスを使用してAI搭載アプリケーションを構築・設定し、DataRobotのコアサービスを利用することができます。 アプリケーションは簡単に共有でき、ユーザーはアプリケーションを使用するための完全なDataRobotライセンスの所有権を取得する必要はありません。 組織でのDataRobot機能の使用を拡大する、優れたソリューションが提供されます。
フライト遅延¶
航空会社はAIを利用して、飛行ルートを最適化し、乗客体験を向上させ、運航を効率化します。 航空機の予防保全から動的な価格設定戦略まで、AIは航空会社がより効率的かつ安全に運用できるようにします。
遅延は、航空会社とその乗客にとって、特にコストがかかることです。 連邦航空局(FAA)によると、米国では2019年に約20%のフライト遅延が発生し、その結果、航空会社、空港、乗客に推定329億ドルの損害が発生しました。 航空会社は遅延を完全に回避することはできませんが、規模を最小限に抑えることで、コストと顧客の不満を抑えることができます。 毎日の出発遅延予測は、遅延が発生する前に、航空交通管制(ATC)、メンテナンス、乗務員の連携、グランドハンドリング、およびスケジューリングの整合性を評価する戦略チームの意思決定プロセスに役立つ可能性があります。
DataRobotには、フライトの遅延を調査するためのユースケースがいくつか用意されています。
- トライアル体験でフライト遅延のガイド付き基本ステップをコードとして適用するには、この AIアクセラレーターを参照してください。
- このデータセットを使用した自己結合を示すコードベースのアプローチについては、 GitHubのAIアクセラレーターを参照してください。
次のような要素でモデリングし、定時運航率(OTP)のAI指標を作成します。
- 定刻通りに出発した便と、出発予定時刻を30分以上過ぎて出発した便の割合。
- 出発空港と到着空港
- 航空会社
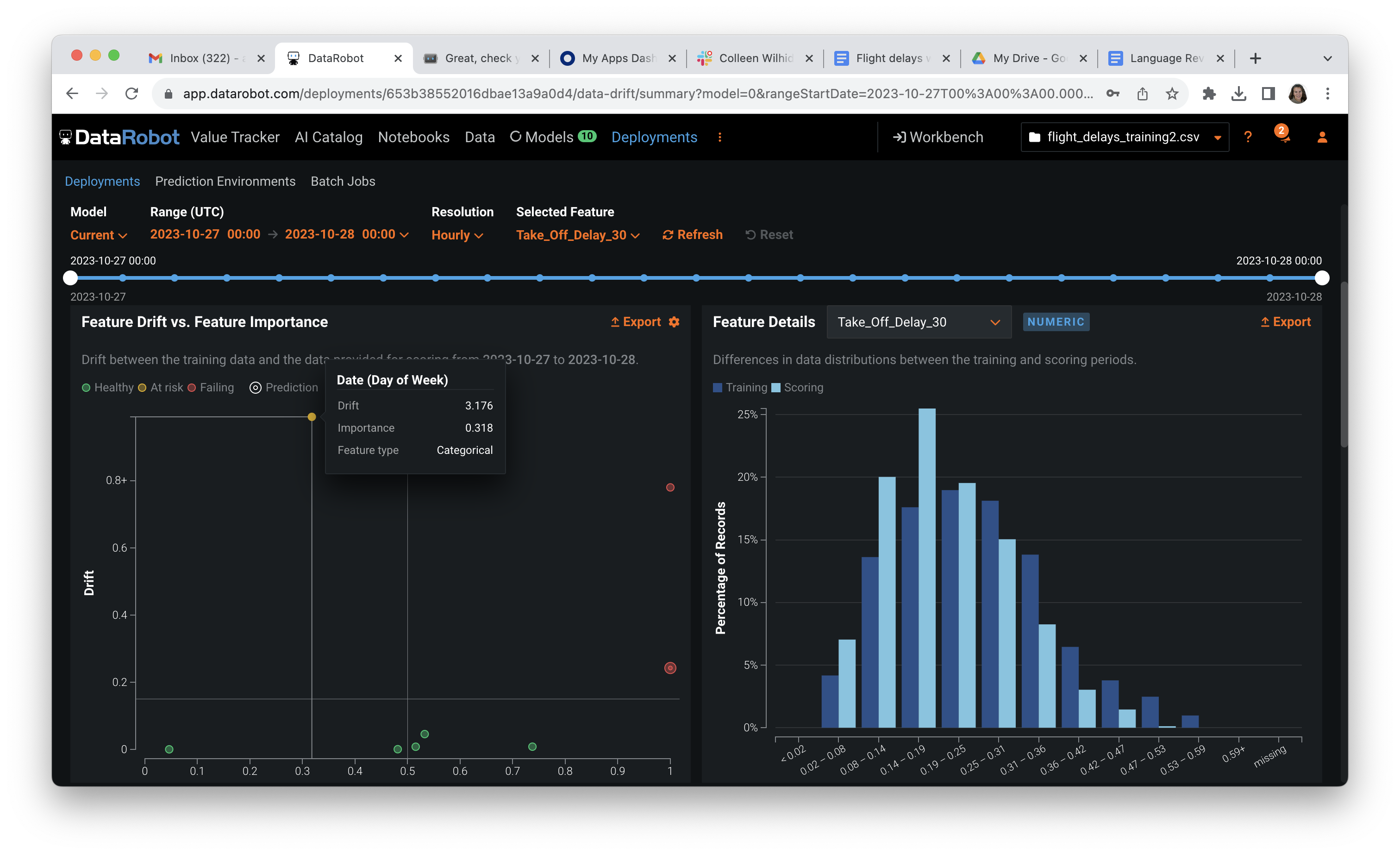
部品故障の予測¶
アバディーングループの調査によると、想定外の機器の故障は1時間あたり26万ドル以上のコストがかかる可能性があり、故障に伴い健康や安全上のリスクが発生する恐れもあります。 定期的な予防保守といった既存のベストプラクティスは、故障を軽減できても、通常とは異なる想定外の故障を検知することはできません。 また、定期的なメンテナンスは、危険なほど保守的になり、過剰なダウンタイムとメンテナンスコストが発生する恐れがあります。 予測モデルは、差し迫った問題が発生する可能性がある場合に、保守担当者に知らせることができます。
このプロアクティブなメンテナンスアプローチ(関連プロセスの自動化)により、オペレーターは次のことが可能になります。
- 収集したセンサーデータから、機器の動作に関する微妙な問題や未知の問題を特定する。
- メンテナンスが本当に必要なときに、メンテナンスをスケジュールする。
- 突然の故障が迫っているときに、対処するよう自動的に通知される。
収集したセンサーデータを活用することで、組織での想定外のダウンタイムを短縮できるだけでなく、機器の故障による意図しない結果を未然に防ぐことができます。
アプリの例:
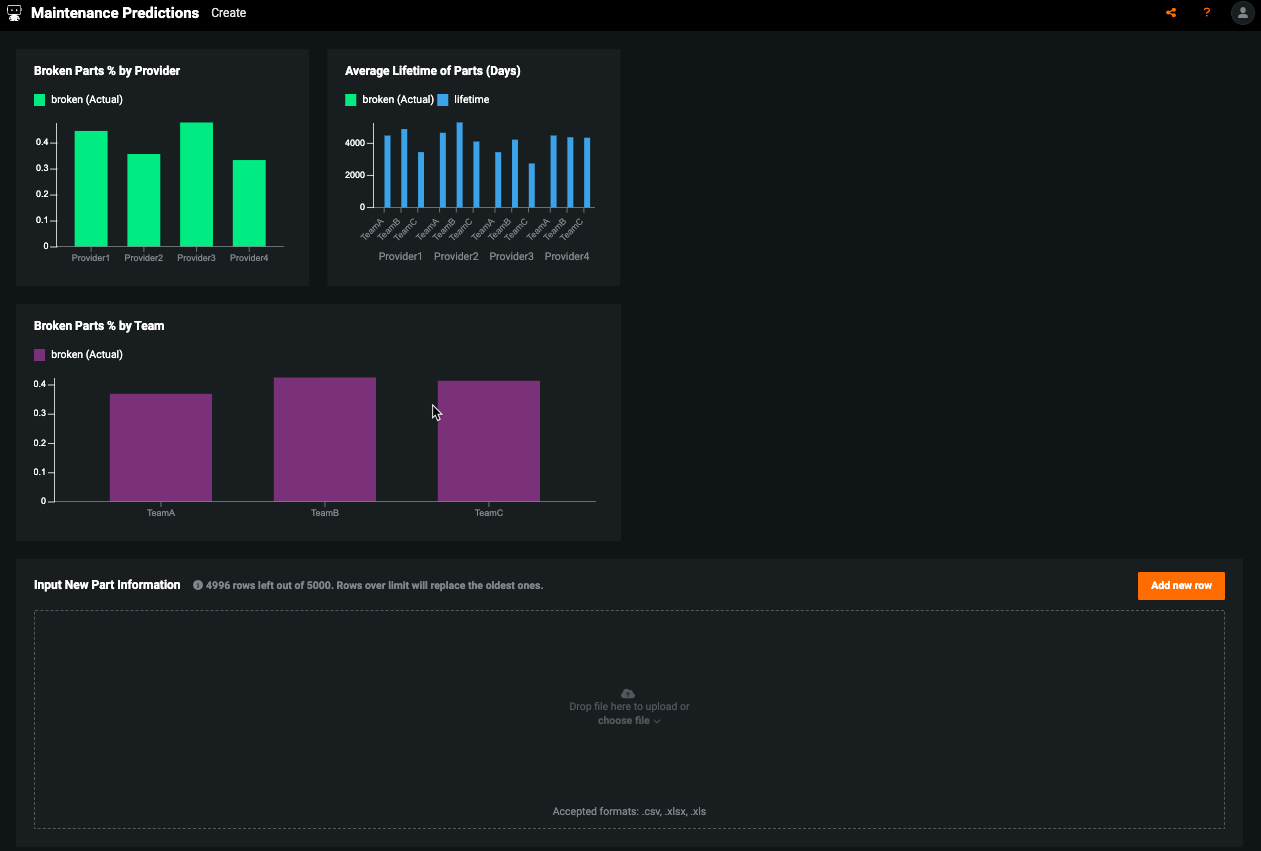
あるいは、 Pythonノートブックを使った予測モデルの構築も検討してみてください。
繰上返済の予測¶
30年の住宅ローンを組んだ場合、通常は30年ぴったりで返済が終わるわけではなく、それより遅くなることも、早くなることもあるし、借り換えをすることもあるでしょう。 規制上の理由および負債の管理のために、銀行はいかなる住宅ローンの有効期限も正確に予測する必要があります。 DataRobotを使用することで、住宅ローンを扱う金融機関は、実務経験とモデリングによるインサイトを組み合わせて、どの住宅ローンが早期に返済される可能性が高いかを把握できます。
一般的な経済データと個人の住宅ローンの記録の間には、ローンの繰上返済を予測する際に利用可能なデータがたくさんあります。 どの特徴量を、どう組み合わせて、どのようなモデリング手法を用いれば、最も精度の高いモデルが得られるかを見極めることが課題です。 さらに、連邦政府の規制では、モデルが差別的でなく堅牢であることを規制当局が検証できるよう、モデルの完全な透明性が求められています。
アプリの例:
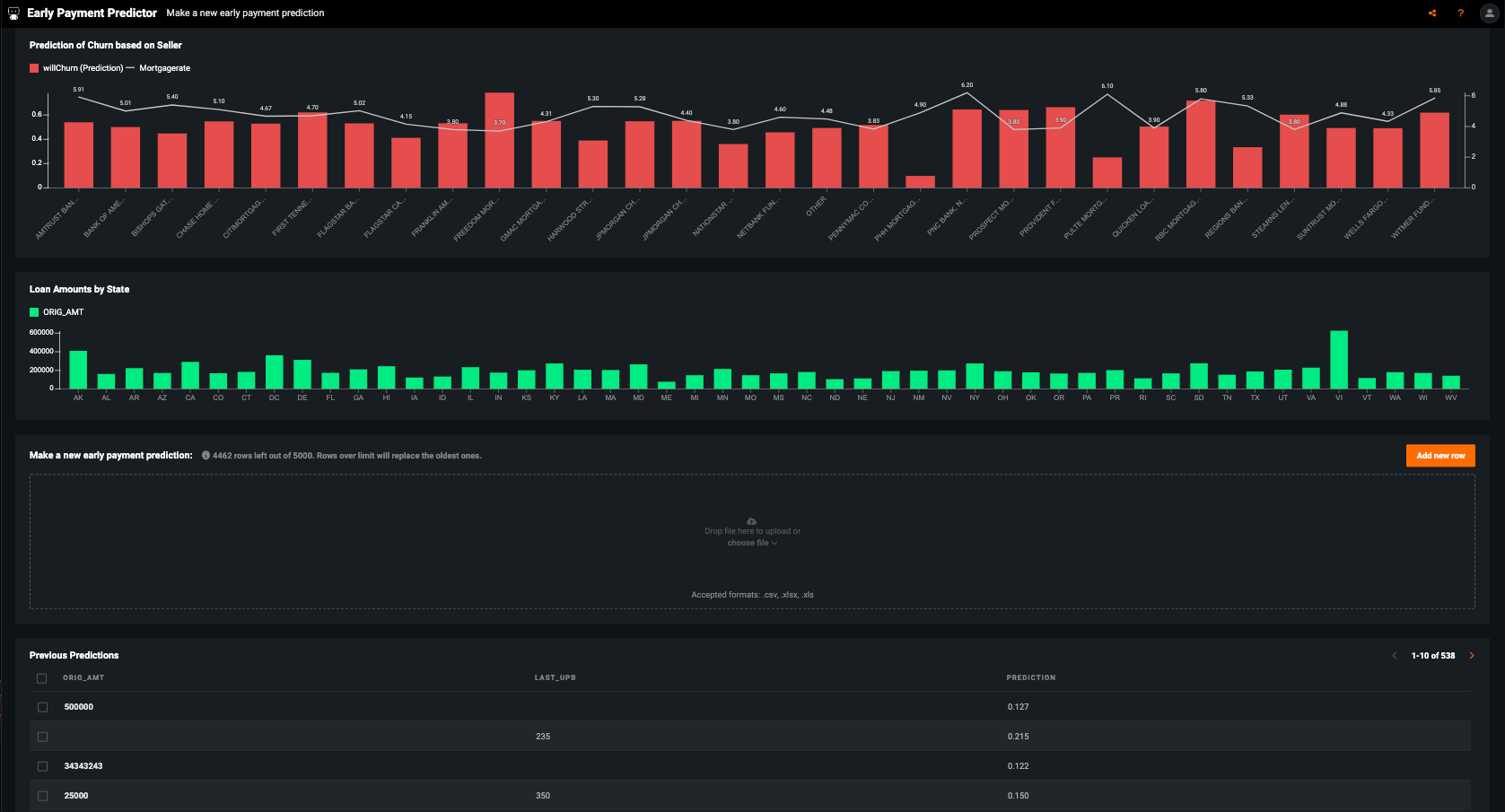
夢の野球チームの予測¶
何百万もの人々が、通常はドラフトまたはオークションベースのリーグでファンタジーベースボールを楽しんでいます。 好きな選手から野球選手のチームを選択するか、平均に対する連続値を考慮せずに昨年の成績に基づいてチームを選択すると、毎年のように比較的弱いチームになる可能性があります。 野球は、統計の観点から、すべてのスポーツの中で最も適切に文書化されているものの1つであり、豊富なデータがあれば機械学習を使用して各選手の本当の才能レベルと翌年の予想される成績について、より良い推定を導き出すことができます。 これにより、より良いドラフトが可能になり、ピークを過ぎた選手への過剰報酬を避けることができます。
ファンタジーベースボールで選手をドラフトする際には、選手のこれまでのキャリアにおける成績や、加齢による影響などの変数を考慮して判断する必要があります。 個人的な解釈で選手の成績を評価すると、直近の成績を過大評価してしまう可能性があります。 つまり、活躍している選手を過大評価したり、活躍していない選手を過小評価したりすることはよくあることです。 過去数年間の成績を考慮して、その選手の翌年の価値をより正確に見積もることを目標とします。 過去の成績から翌年の成績を予測する機械学習モデルを構築すれば、好不調が偶然である場合と、その選手の今後の成績の指標となる場合を識別できるようになります。
アプリの例:
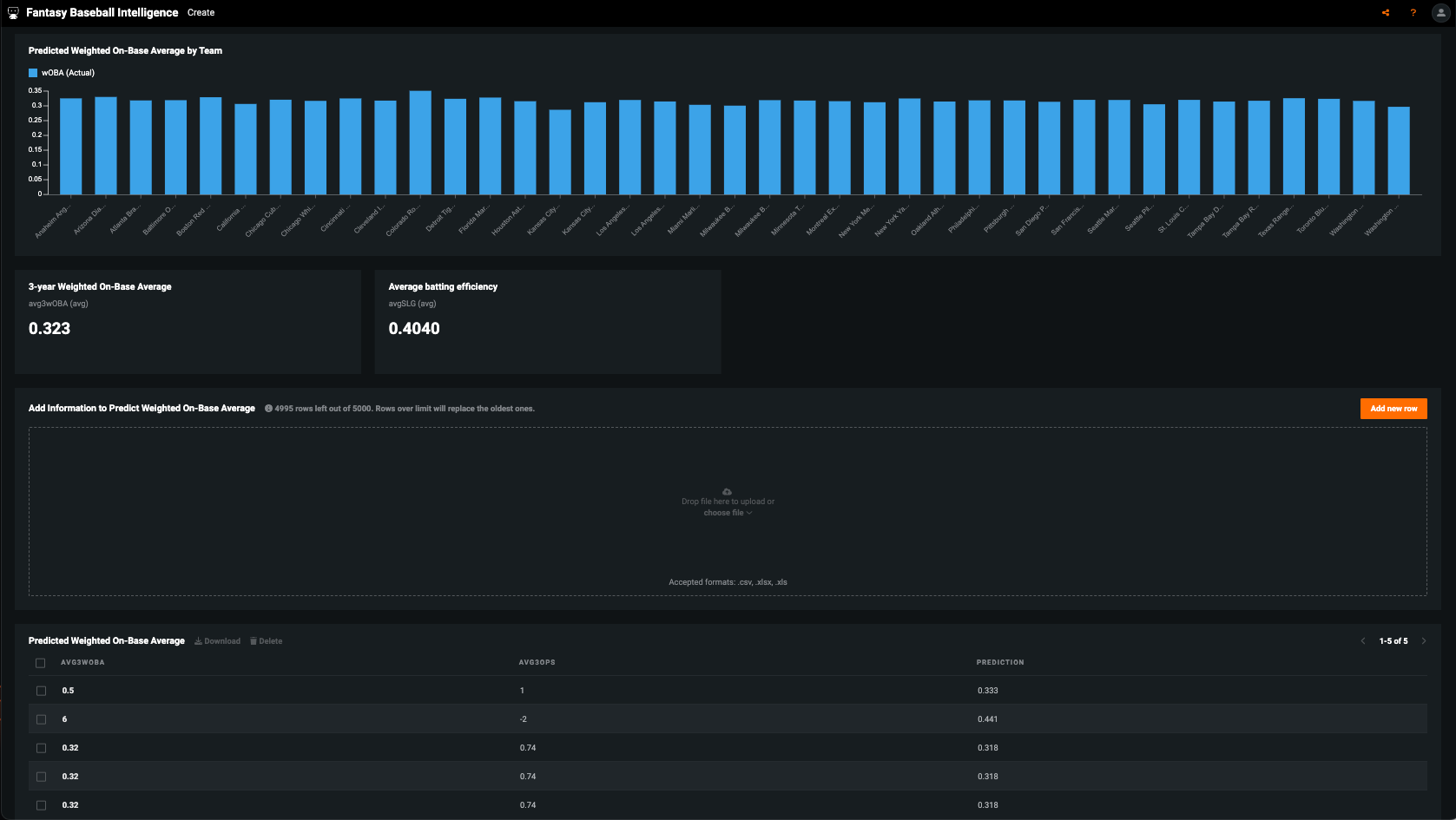
あるいは、 Pythonノートブックを使った予測モデルの構築も検討してみてください。