AIプラットフォームリリース¶
DataRobotのマネージドAIプラットフォーム向けに毎月発表されているプレビューと一般提供の新機能を記録しています。 サポート終了のお知らせも含まれており、必要に応じて、サポート終了ガイドにリンクしています。
2月にリリースされたSaaS機能のお知らせ¶
2025年2月
このページでは、2025年2月に新たにリリースされ、DataRobotのSaaS型マルチテナントAIプラットフォームで利用できる機能についてのお知らせと、追加情報へのリンクを掲載しています。 リリースセンターからは、過去にリリースされた機能のお知らせや、セルフマネージドAIプラットフォームのリリースノートにもアクセスできます。
2月リリースの機能¶
次の表は、新機能の一覧です。
目的別にグループ化された機能
名前 | NextGen | Classic |
---|---|---|
データ | ||
レジストリでのNextGenデータアセットの管理 | ✔ | |
ワークベンチでのデータ準備をさらに改善 | ✔ | |
モデリング | ||
時間認識データラングリングを一般提供 | ✔ | |
時系列エクスペリメントでユニバーサルSHAPのサポートを開始 | ✔ | |
Composable MLにスパース性関連のタスクを追加 | ✔ | ✔ |
単一ビューでのモデル比較機能を一般提供 | ✔ | |
Classicでのブループリント詳細ビューを一般提供 | ✔ | |
予測とMLOps | ||
レジストリでモデルのインサイトを表示 | ✔ | |
実行環境の改善(一般提供) | ✔ | |
デプロイで予測警告を有効にする | ✔ | ✔ |
アプリケーション | ||
カスタムアプリケーションでPython以外の依存関係をインストールする | ✔ | |
「データと会話する」エージェントのアプリケーションテンプレート | ✔ |
データ¶
レジストリでのNextGenデータアセットの管理¶
レジストリのデータページは、NextGenでデータセットを管理するための一元的なハブであり、データを簡単に検索、共有、探索、再利用することができます。 レジストリに直接追加したデータセット、ユースケースにリンクしたデータセット、他のユーザーと共有したデータセット、または自分がメンバーになっているユースケースに他のユーザーが追加したデータセットがここに表示されます。 データレジストリでは、セキュリティ、コンプライアンス、一貫性を確保しながら、ビジネス上の問題への対処に必要なデータに簡単にアクセスできます。
データレジストリにアクセスするには、NextGenでレジストリを開き、データをクリックします。 ここから、データの確認、共有、削除ができます。
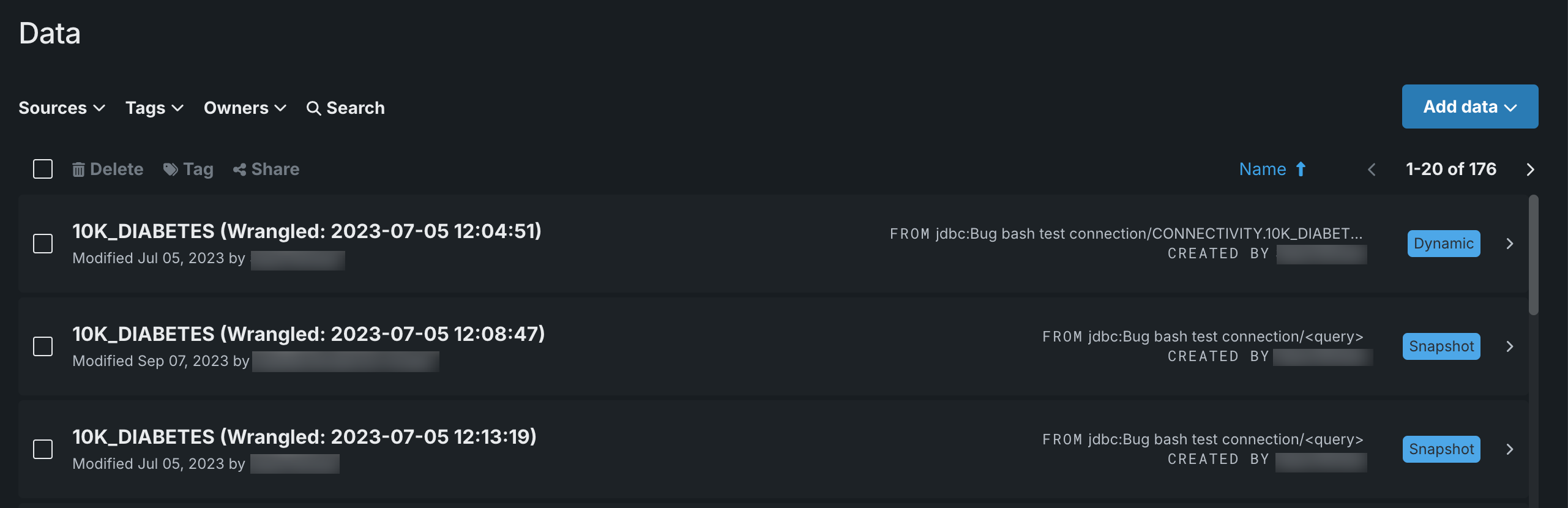
次に、個々のデータセットをクリックすると、データセットのプレビュー、メタデータ、インサイト、バージョン履歴、関連アクティビティが表示されます。
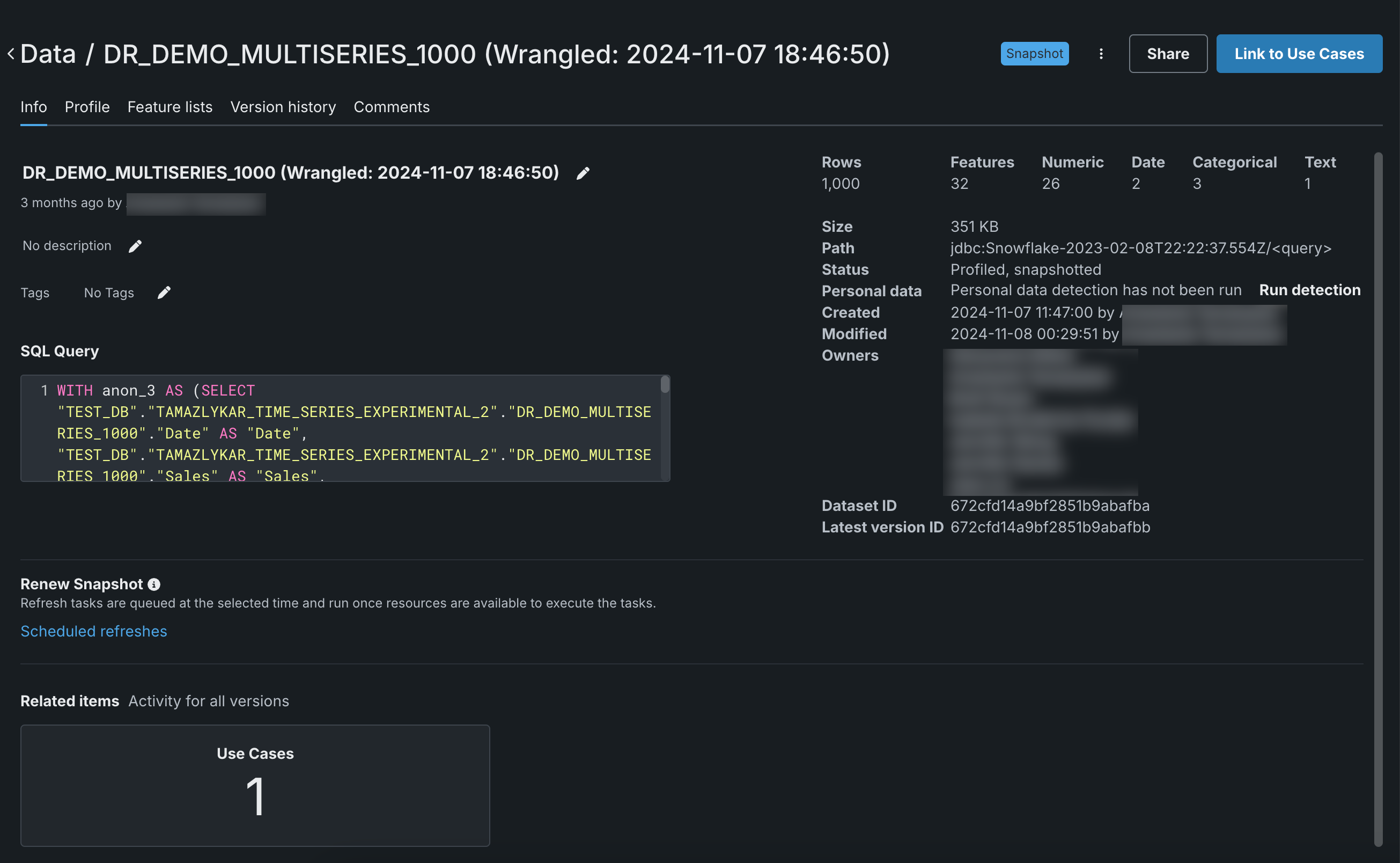
ワークベンチでのデータ準備をさらに改善¶
このリリースでは、ワークベンチのデータ準備機能が以下のようにアップデートされました。
-
ユースケースにデータを追加する前に、データをキャンバスに直接ドラッグアンドドロップしたり、提供されている別のアップロードオプションを選択したりできます。
-
データを追加モーダルでは、データをドラッグアンドドロップしてデータレジストリに登録することができます。
-
URLを使ってデータを追加することもできるようになりました。
モデリング¶
時間認識データラングリングを一般提供¶
時間認識ラングリングを使えば、時間認識データの操作レシピを作成し、データ準備段階で時系列特徴量エンジニアリングを実行できます。 この方法では、時間認識ユースケースにおいて、10GBを超えるデータセットに特徴量エンジニアリングのメリットを活かします。 一般提供版では、Snowflake、Databricks、BigQueryの接続をサポートしています。 Postgresの接続とDataRobotのデータレジストリデータセットは現在プレビュー機能です。 ユーザー定義関数のインターフェイスが改善されたことで、新しい関数の作成や保存済みの関数の使用が可能になり、クエリーのパフォーマンスを大幅に向上させることができます。
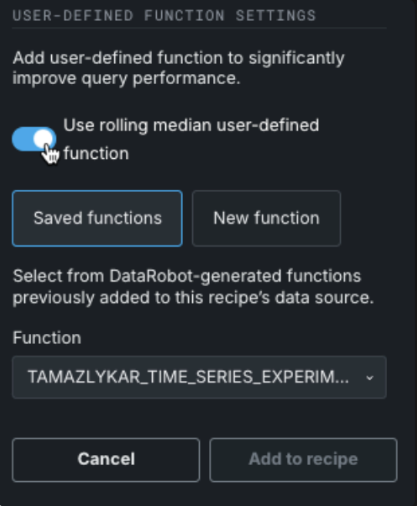
時系列エクスペリメントでユニバーサルSHAPのサポートを開始¶
今回のデプロイから、ワークベンチで時系列インサイト(特徴量のインパクト、個々の予測の説明、SHAP分布:特徴量ごと)のためにSHAP値の計算が可能になりました。 時系列エクスペリメントのモデルの場合、プライマリー日付、予測距離、および系列ID(存在する場合)の組み合わせごとに一意のSHAP値セットが計算されます。 すべての予測距離が考慮されます。 ドロップダウンを使用して、表示を制御します。
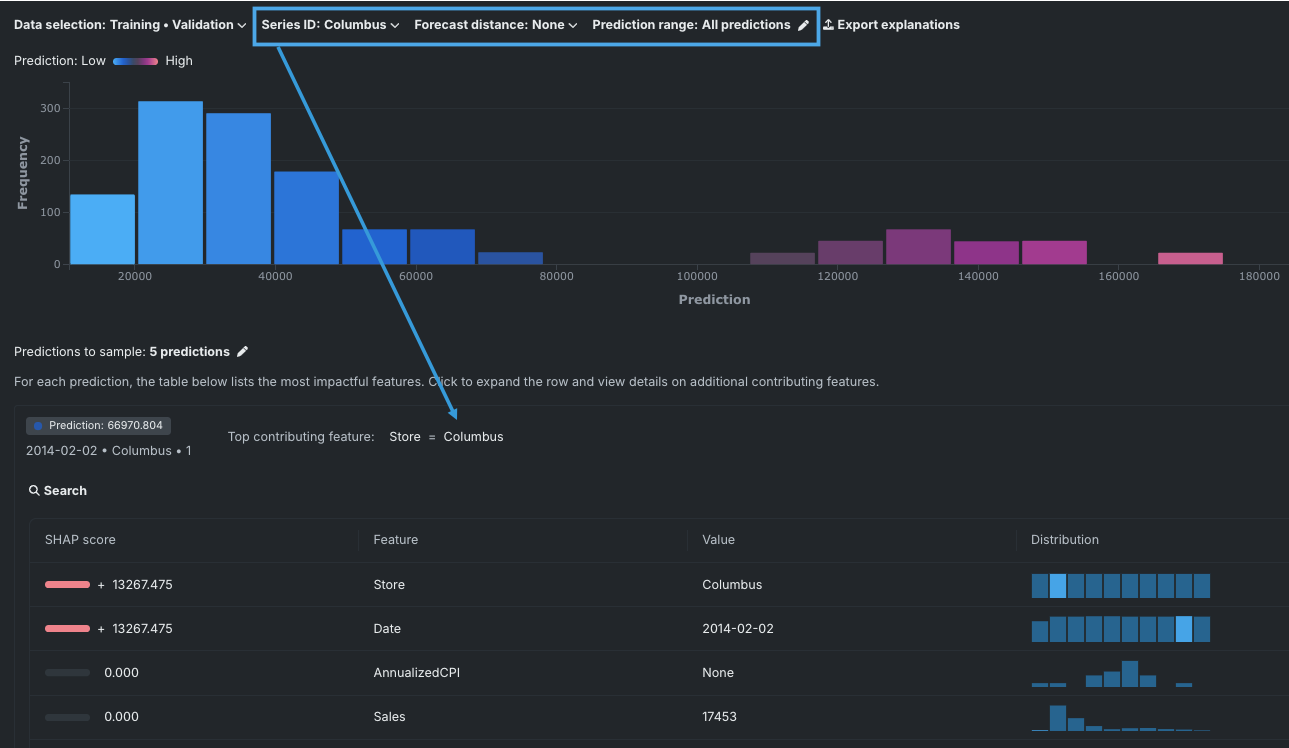
Composable MLにスパース性関連のタスクを追加¶
Composable MLでは、スパース性に関する特定の入力要件を持つタスクがあります。 互換性を高め、これらのタイプのダウンストリームタスクに簡単に接続できるようにするため、2つの新しいタスク(Sparse to DenseとDense to Sparse)を使用して、カスタムコードなしで変換を実行できるようになりました。
単一ビューでのモデル比較機能を一般提供¶
2023年9月にプレビュー機能としてリリースされたワークベンチのモデル比較機能が、時間認識以外のエクスペリメント(二値分類、連続値)で一般提供機能になりました。 機械学習でビジネス上の問題を解決する反復プロセスを簡略化するため、ワークベンチにはモデル比較ツールが用意されており、1つのユースケース内の任意の数のエクスペリメントから最大3つのモデルを並べて比較できます。 各エクスペリメントを個別に確認し、後で比較するために指標を記録する代わりに、単一のビューでさまざまなエクスペリメントのモデルを比較できます。
比較リーダーボードは、ワークベンチ内の任意のプロジェクトからアクセスできます。 フィルターを使用して、モデルの検索と選択、さまざまなインサイトでのモデルの比較、選択したモデルのメタデータの表示と比較を容易に行うことができます。 デモ動画をご覧ください。
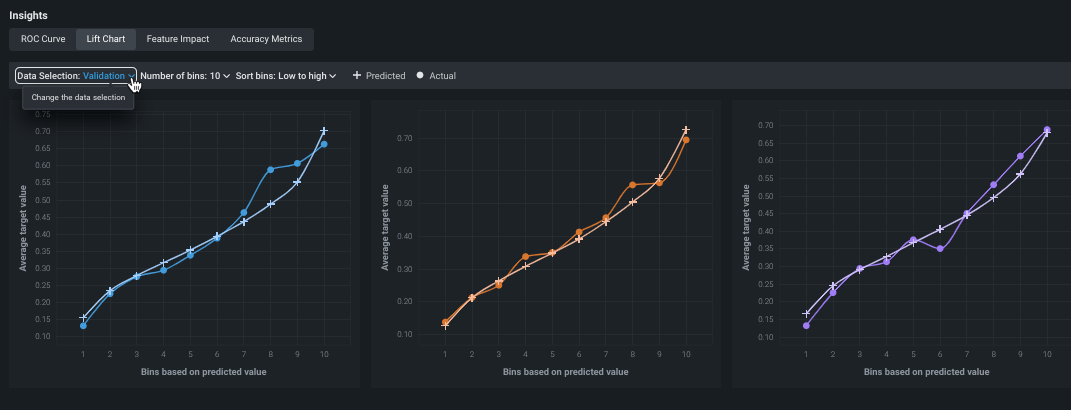
Classicでのブループリント詳細ビューを一般提供¶
リーダーボードのブループリントタブで確認できるブループリントは、デフォルトでは読み取り専用の要約ビューであり、最終モデルで使用されたタスクのみが表示されます。 しかし、元のモデリングアルゴリズムにはさらに多くの「ブランチ」が含まれていることが多く、DataRobotは、プロジェクトデータや特徴量セットに適用できないブランチを削除しています。 今回のリリースから、読み取り専用モードで詳細ビューの表示に切り替えられるようになりました。 以前はプレビュー機能であり、機能フラグが必要でした。 今回から一般提供機能になりました。
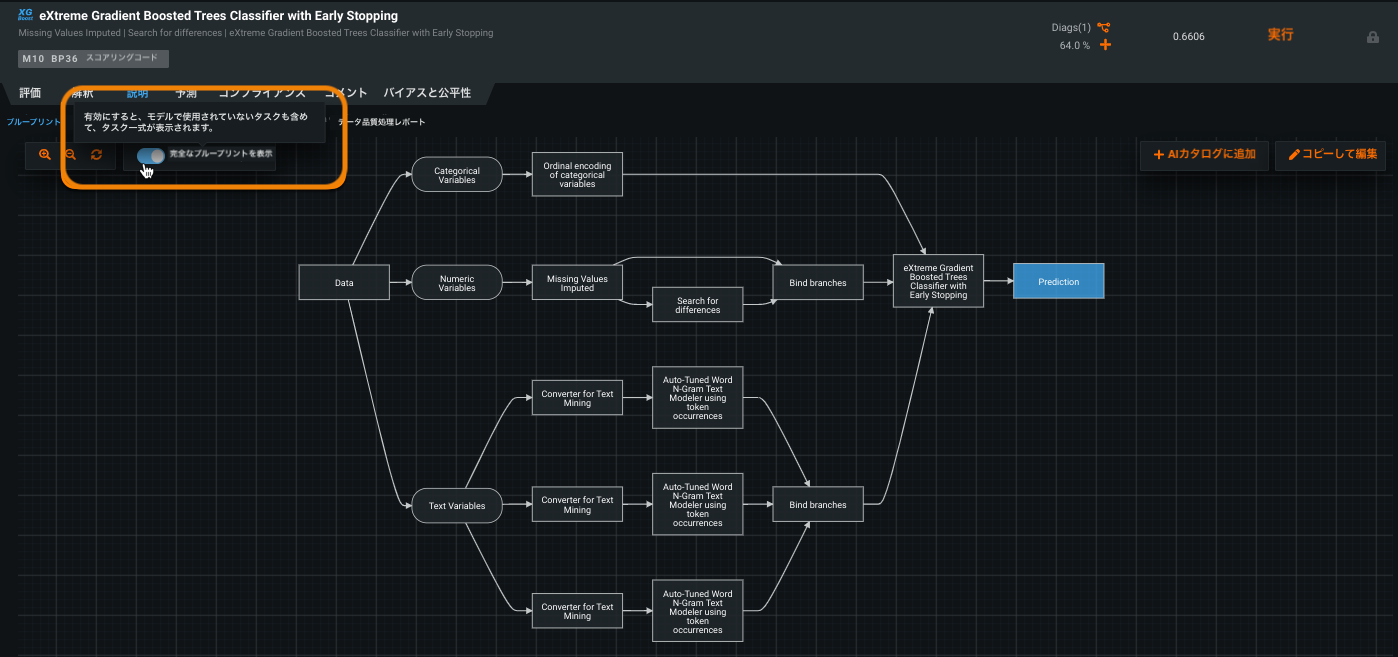
予測とMLOps¶
レジストリでモデルのインサイトを表示¶
レジストリ内のDataRobotモデルとカスタムモデルのインサイトタブには、特徴量のインパクトに加えて、個々の予測の説明とSHAP分布:特徴量ごとが含まれるようになりました。 これらのインサイトは、二値分類と連続値の問題タイプでサポートされています。
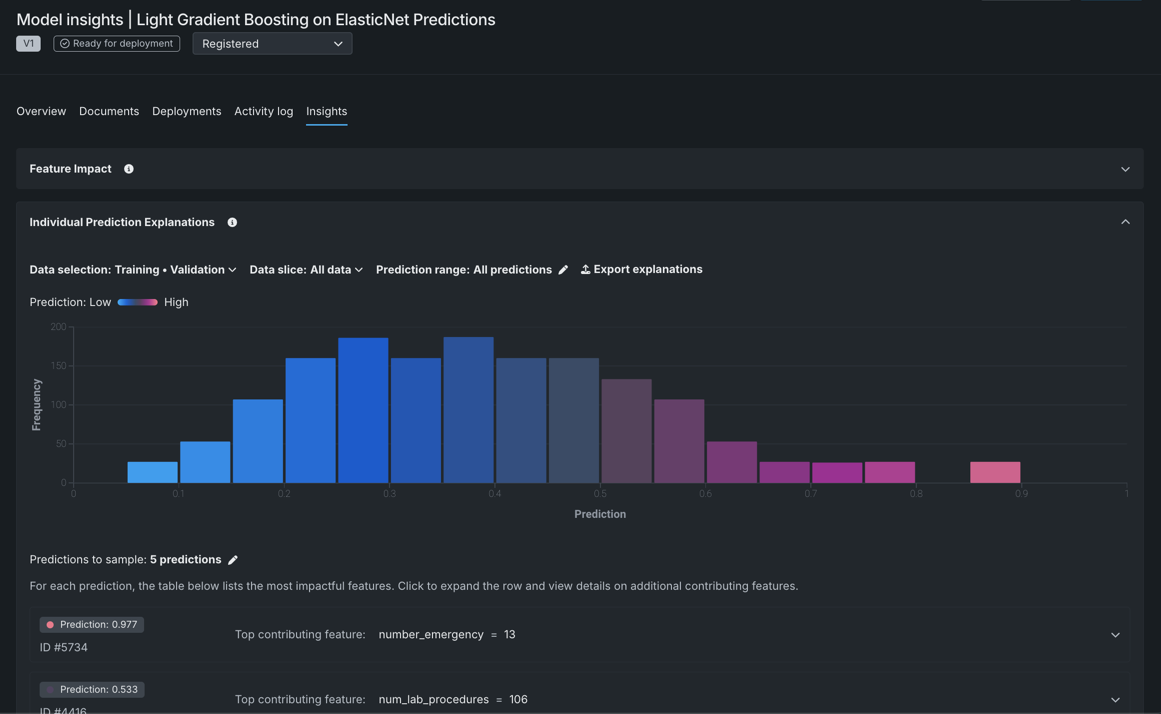
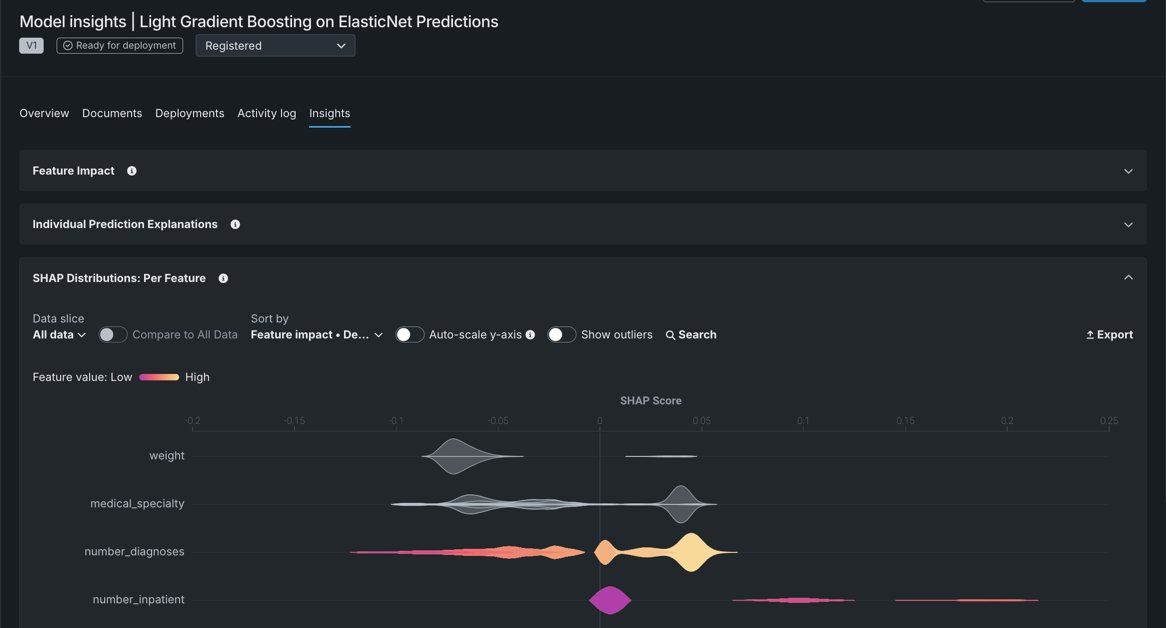
実行環境の改善(一般提供)¶
カスタムモデルを作成して環境を選択したら、環境バージョンを管理して最新の状態にすることができます。 更新したいモデルとバージョンについて、アセンブルタブで環境セクションに移動します。 環境バージョンメニューで、より新しいバージョンの環境が利用可能な場合は、最新のものを使用をクリックしてカスタムモデルを更新し、正常に構築された最新バージョンを使用することができます。
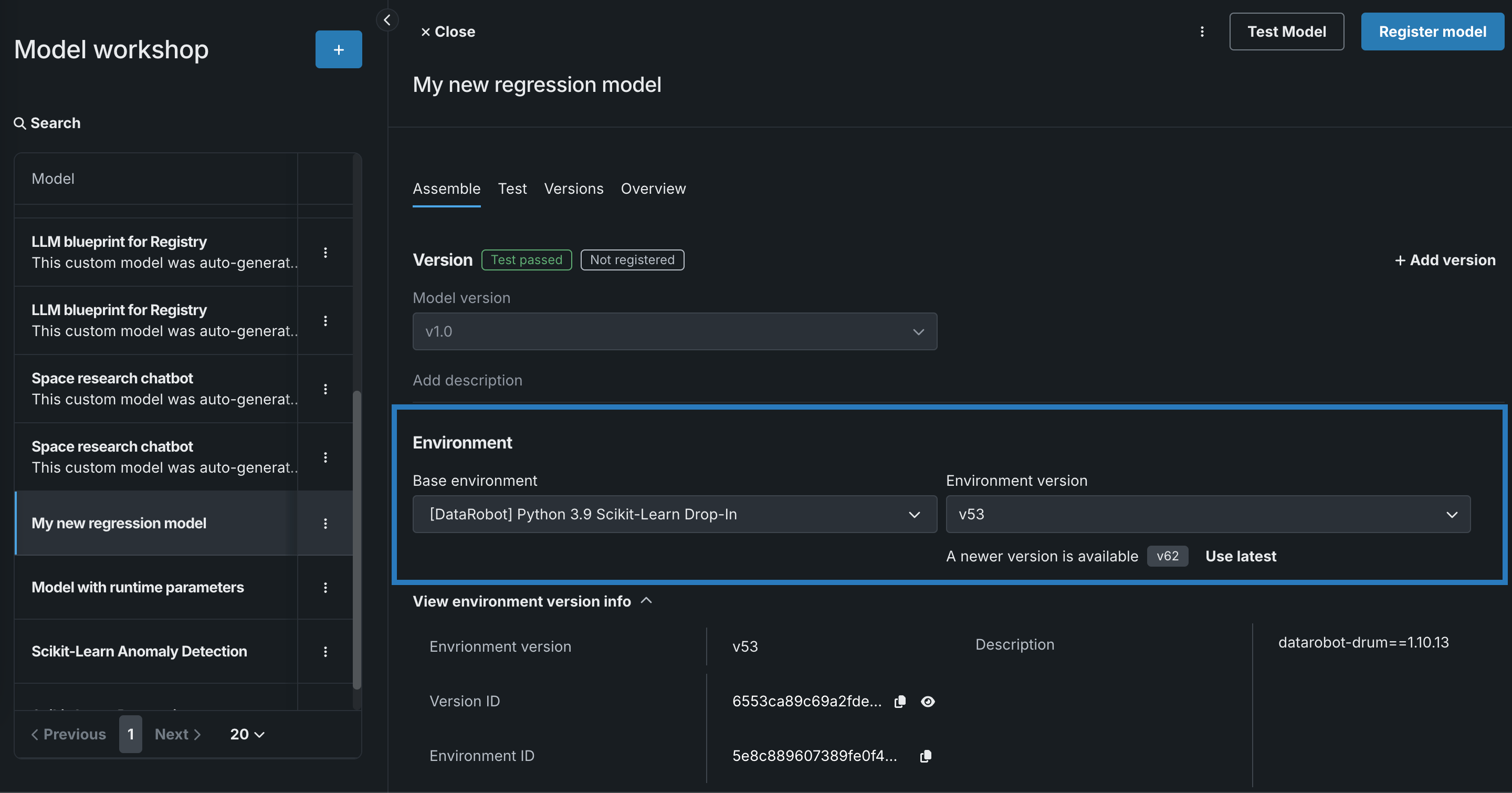
さらに、環境バージョンの情報を表示をクリックすると、環境のバージョン、バージョンID、環境ID、説明を確認できます。
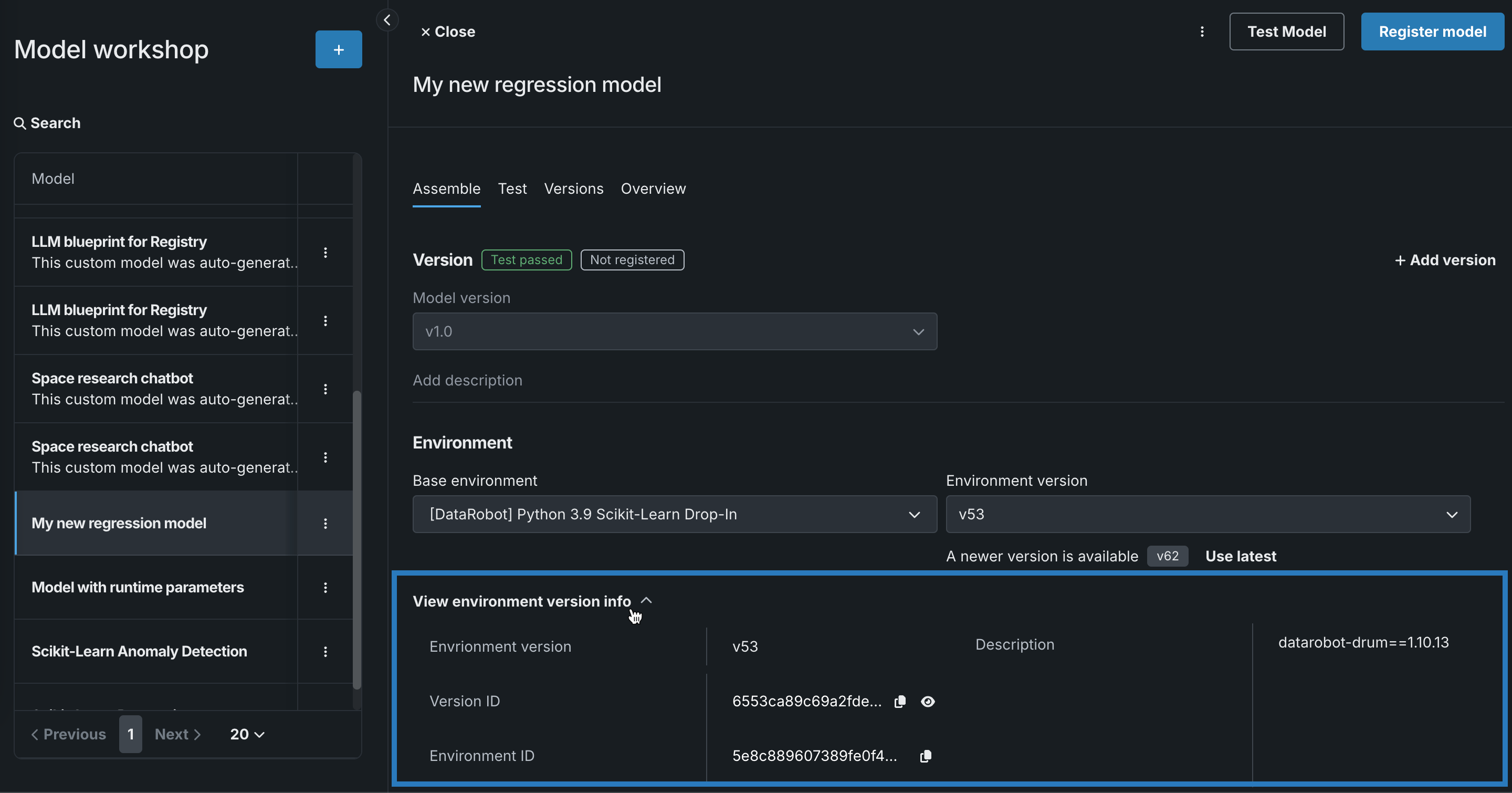
カスタム環境のバージョン情報は、カスタムモデルのバージョン詳細でも確認できます。
デプロイで予測警告を有効にする¶
信頼性 > 予測警告タブで、連続値モデルのデプロイに対して予測警告を有効にします。 予測警告を使用すると、予期された結果に予測が一致しないときを識別することによって、運用環境でのリスクを緩和し、モデルをより頑強にできます。 この機能では、いつ外れ値を含む予測がデプロイで生成されるかが検出され、その結果が予測と共にまとめてレポートされます。
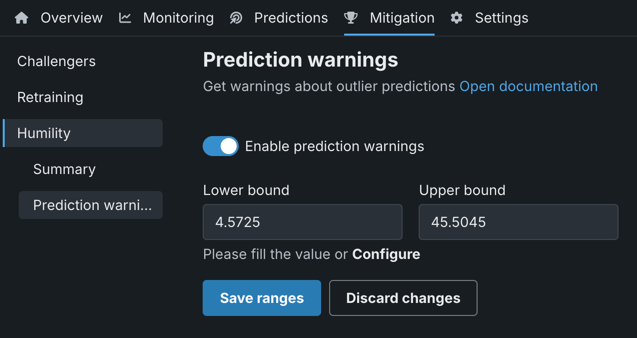
デプロイで予測警告を有効にすると、警告をトリガーする異常な予測値には、時間経過に伴う予測の棒グラフでフラグが設定されます。 棒グラフの黄色のセクションは、ある時点における異常な予測を表しています。 特定の期間の異常な予測の数を表示するには、棒グラフのフラグ付き予測に対応するプロット上のポイントにカーソルを合わせます。
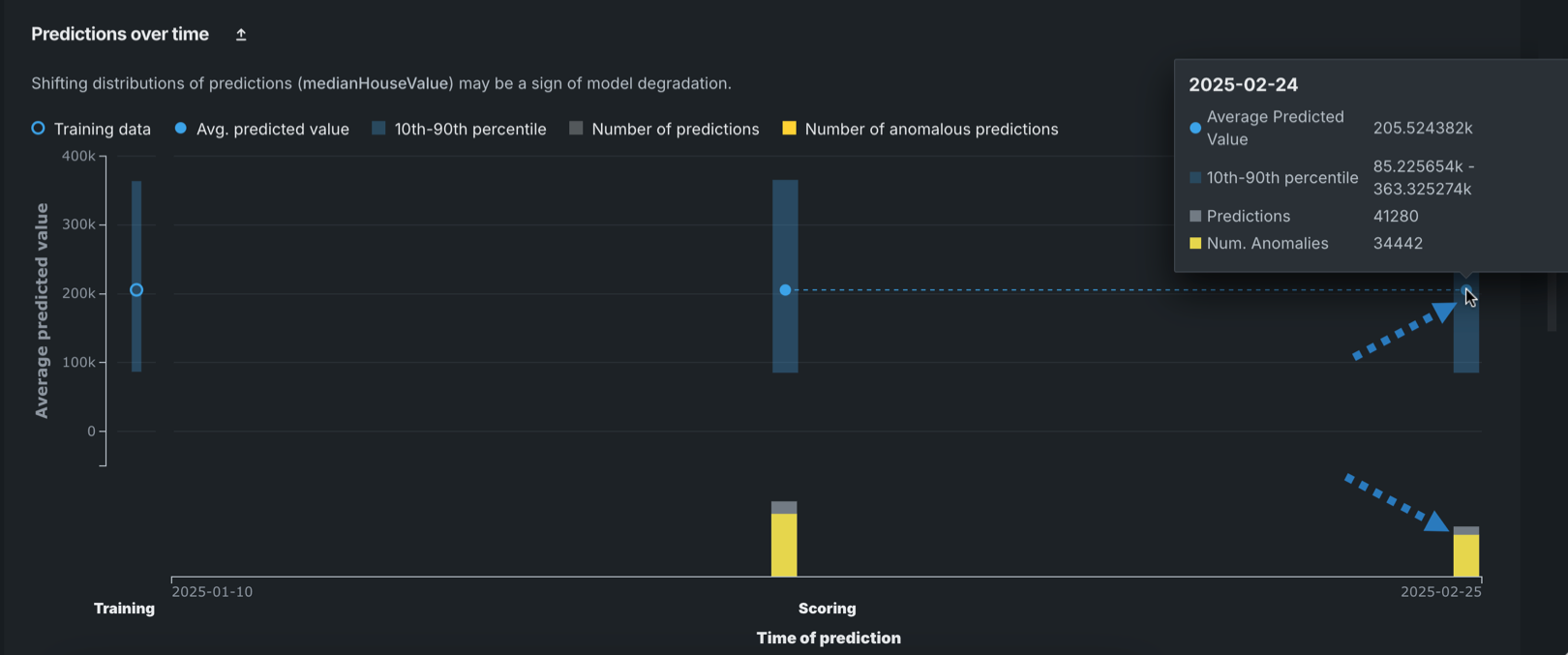
アプリケーション¶
「データと会話する」エージェントのアプリケーションテンプレート¶
「データと会話する」エージェントのアプリケーションテンプレートを利用すると、エージェントワークフローを使って、.csvまたはデータベースの表形式および構造化データに関する質問ができます。 このアプリケーションでは、チャットインターフェイスを介してデータのアップロードまたは接続、質問、インサイトによる回答の視覚化を行い、複雑なデータセットから迅速にインサイトを得ることができます。
意思決定者はデータに基づくインサイトを頼りにしていますが、インサイトを得るまでに時間と労力がかかることに不満を感じることがよくあります。 彼らは簡単な質問への回答を待つことを嫌い、このフラストレーションを解消するソリューションには多額の投資を惜しみません。 このアプリケーションは、スプレッドシートやデータベースへの平易な言語によるチャットインターフェイスを提供することで、この課題に直接対処します。 直感的な会話を通じて、元のデータを実用的なインサイトに変換します。 AIの能力を活用することで、より迅速な分析が可能となり、より短時間で情報に基づいた意思決定を行うことができます。
カスタムアプリケーションでPython以外の依存関係をインストールする¶
カスタムアプリケーションを構築する際、アプリケーションソースにrequirements.txt
ファイルを提供することで、アプリ構築時にPythonの依存関係をインストールするよう指示することができます。 Python以外の依存関係をインストールするために、アプリケーションソースの一部としてbuild-app.sh
スクリプトを提供できるようになりました。 このスクリプトは、初めてアプリケーションを構築するときに呼び出されます。 build-app.sh
スクリプトはnpm install
またはyarn build
を実行できるため、カスタムアプリケーションはJavaScriptベースのアプリケーションでの依存関係のインストールに対応できます。