MLOps and predictions (V10.0)¶
April 29, 2024
The DataRobot MLOps v10.0 release includes many new features and capabilities, described below. See additional details of Release 10.0 in the data and modeling and code-first release announcements.
New features and enhancements¶
Features grouped by capability
* Premium feature
In the spotlight¶
Updated layout for the NextGen Console¶
This update to the NextGen Console provides important monitoring, predictions, and mitigation features in a modern user interface with a new and intuitive layout.
Video: NextGen Console
This updated layout provides a seamless transition from model experimentation in Workbench and registration in Registry, to model monitoring and management in Console—while maintaining the features and functionality available in DataRobot Classic.
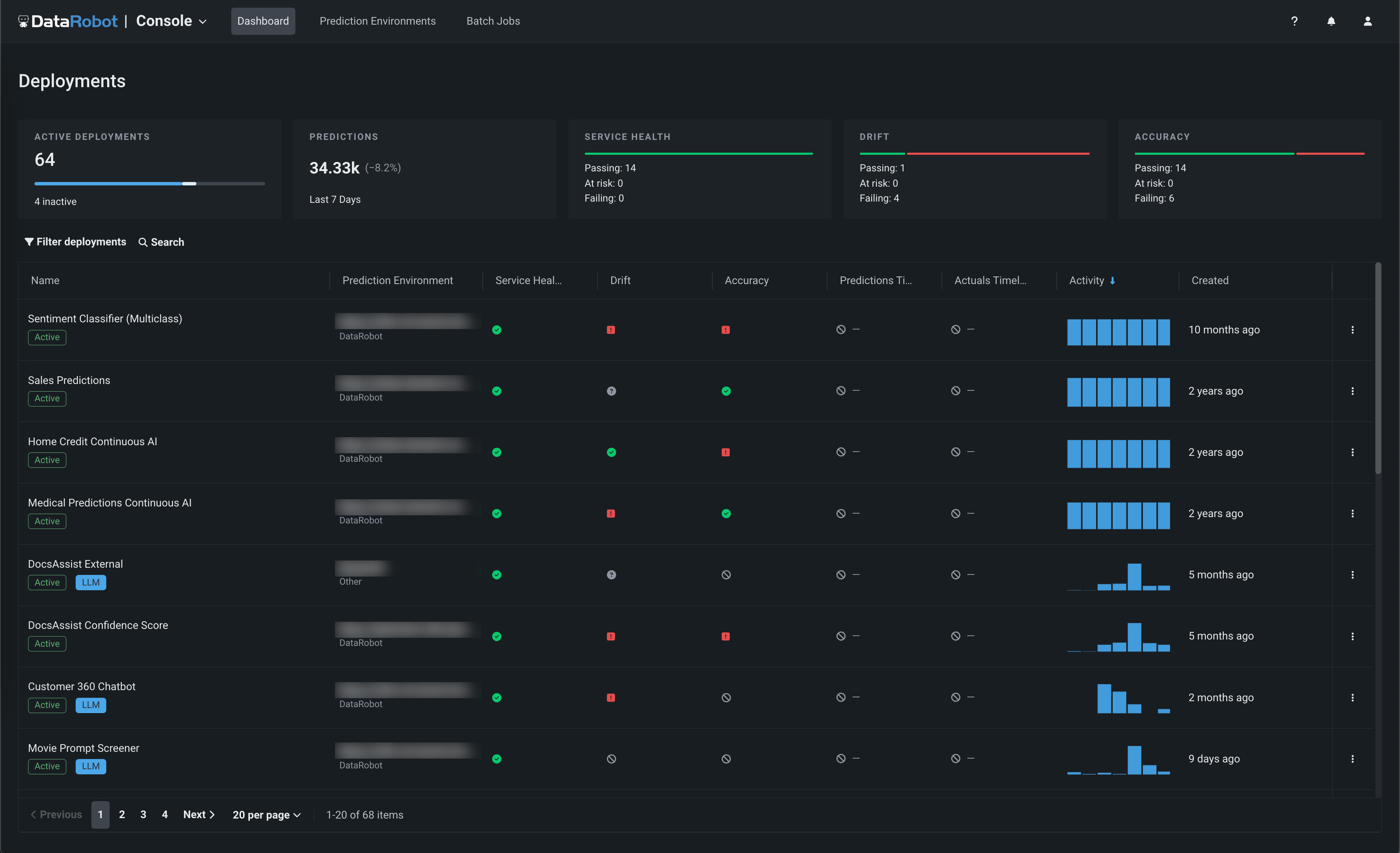
For more information, see the documentation.
NextGen Registry GA¶
Now generally available in the NextGen Experience, the Registry is an organizational hub for the variety of models used in DataRobot. The Registry > Model directory page lists registered models, each containing deployment-ready model packages as versions. These registered models can contain DataRobot, custom, and external models as versions, allowing you to track the evolution of your predictive and generative models and providing centralized management:
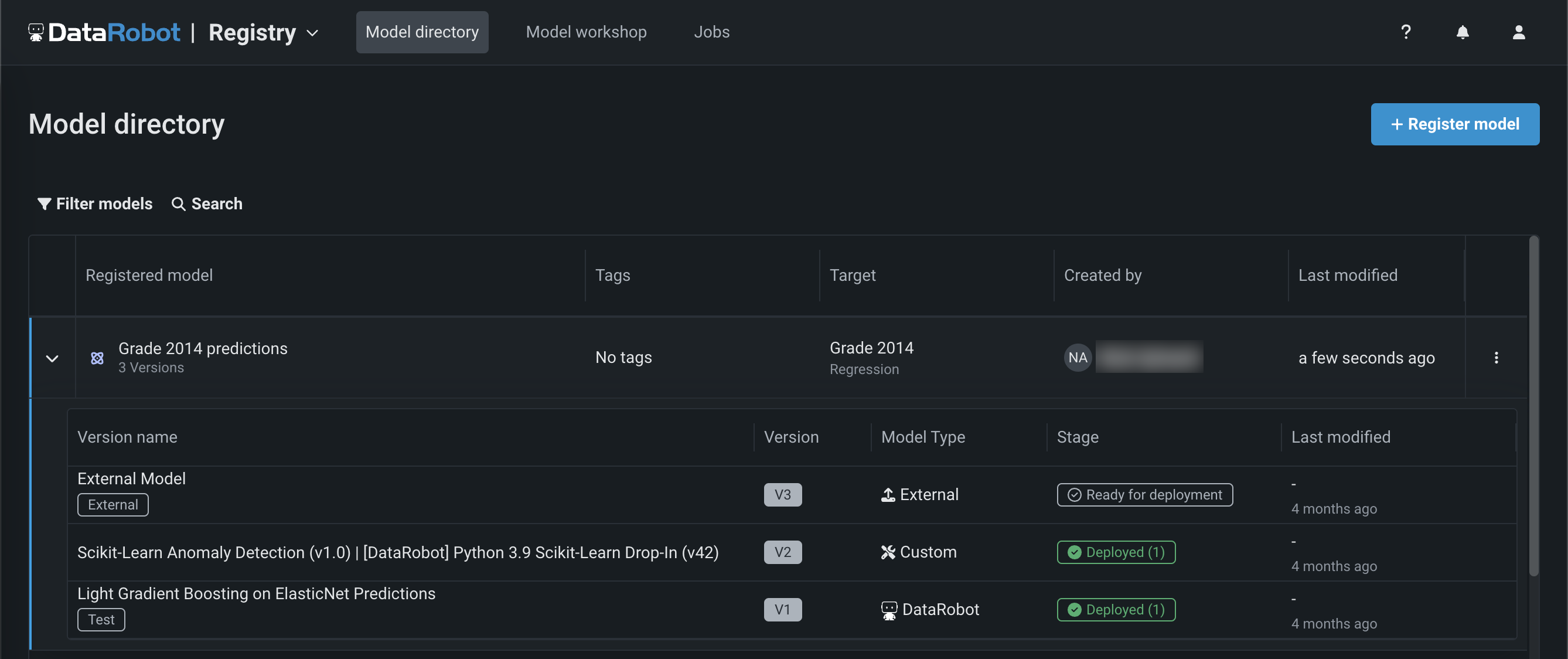
With this release, the registry tracks the System stage and the configurable User stage of a registered model version. Changes to the registered model version stage generate system events. These events can be tracked with notification policies:
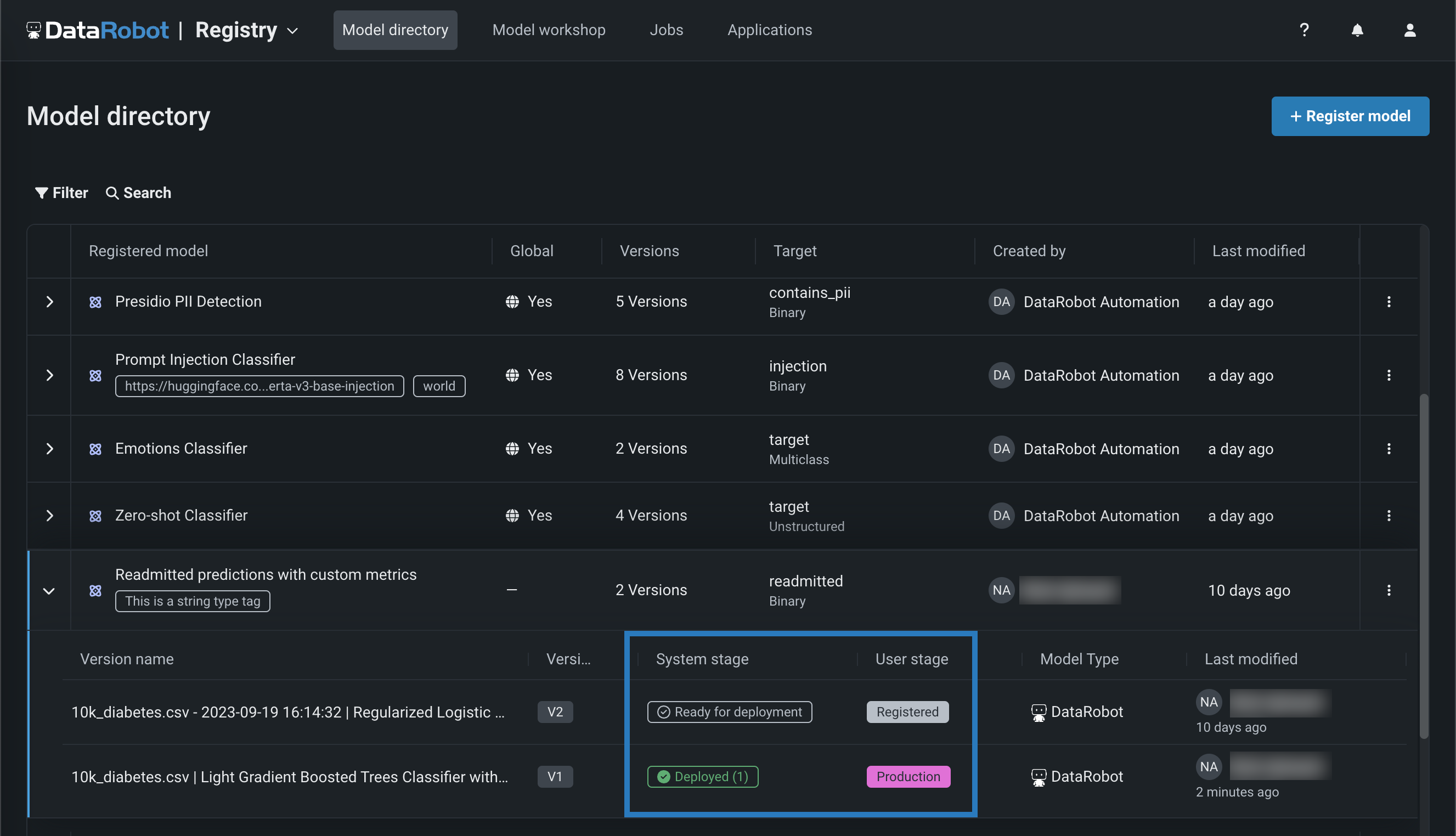
The Registry > Model workshop page allows you to upload model artifacts to create, test, register, and deploy custom models to a centralized model management and deployment hub. Custom models are pre-trained, user-defined models that support most of DataRobot's MLOps features. DataRobot supports custom models built in a variety of languages, including Python, R, and Java. If you've created a model outside of DataRobot and want to upload your model to DataRobot, define the model content and the model environment in the model workshop:
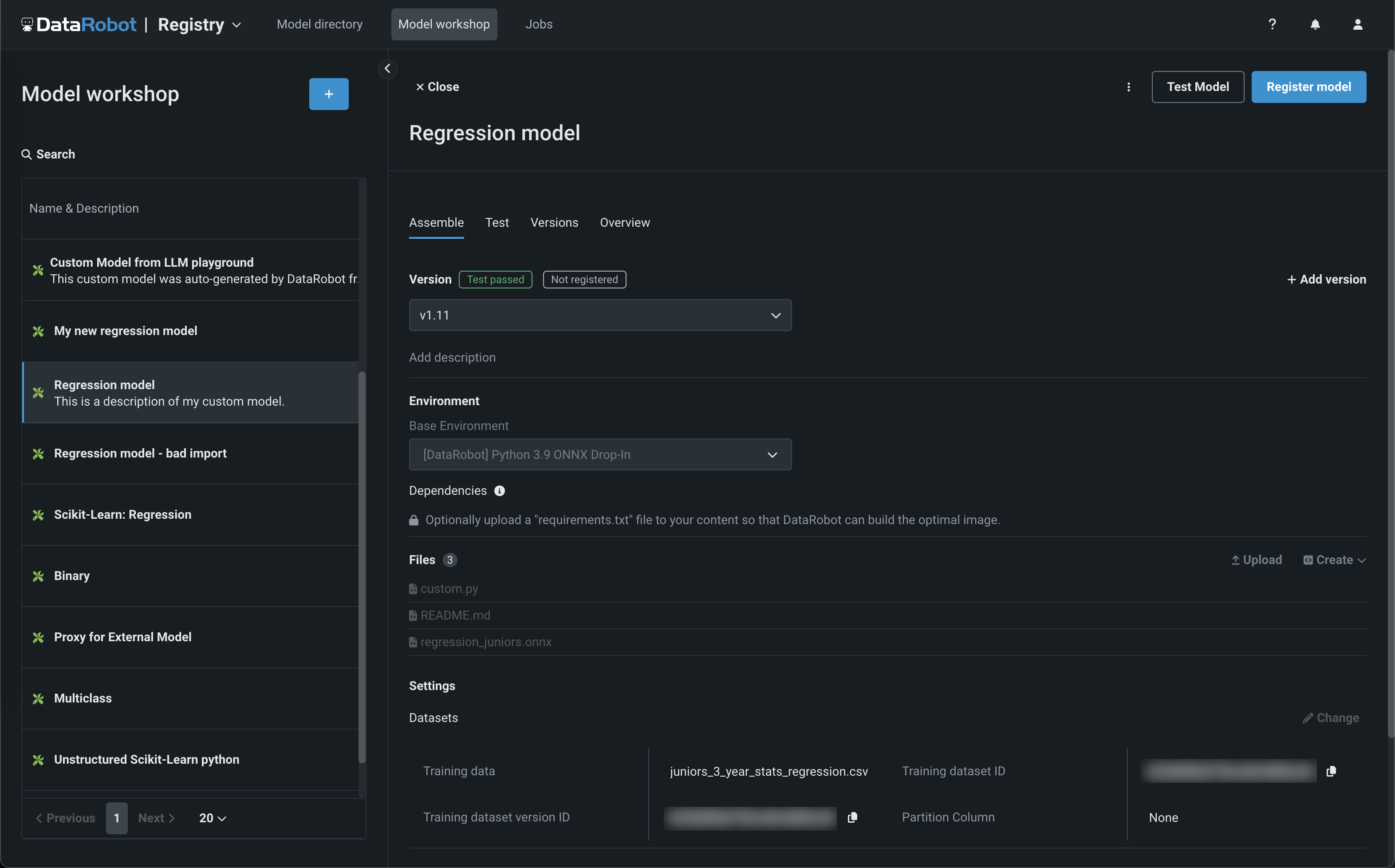
The Registry > Jobs page uses jobs to implement automation (for example, custom tests, metrics, or notifications) for models and deployments. Each job serves as an automated workload, and the exit code determines if it passed or failed. You can run the custom jobs you create for one or more models or deployments. The automated workloads defined through custom jobs can make prediction requests, fetch inputs, and store outputs using DataRobot's Public API:
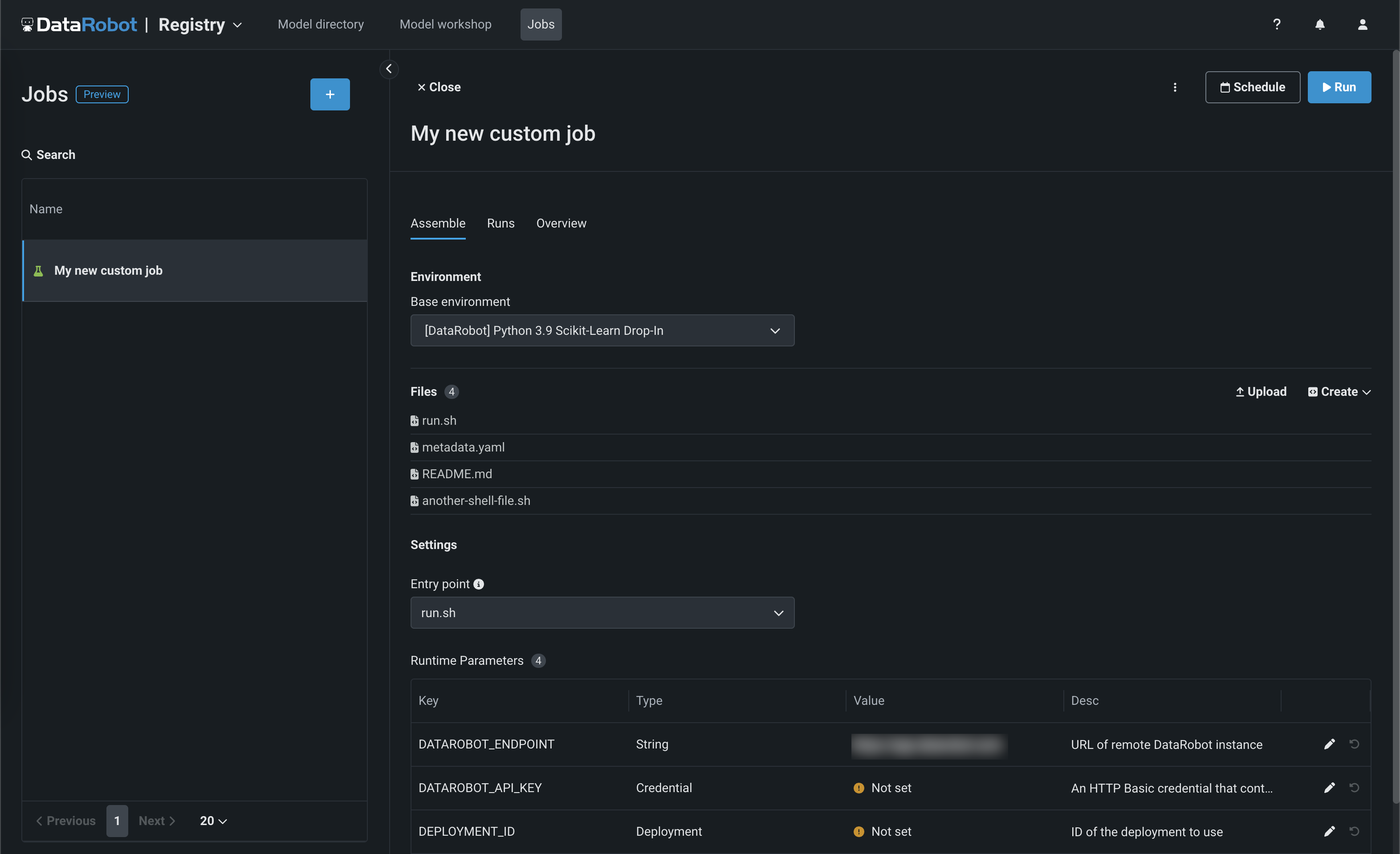
With this release, custom jobs include a Resources settings section where you can configure the resources the custom job uses to run and the egress traffic of the custom job:
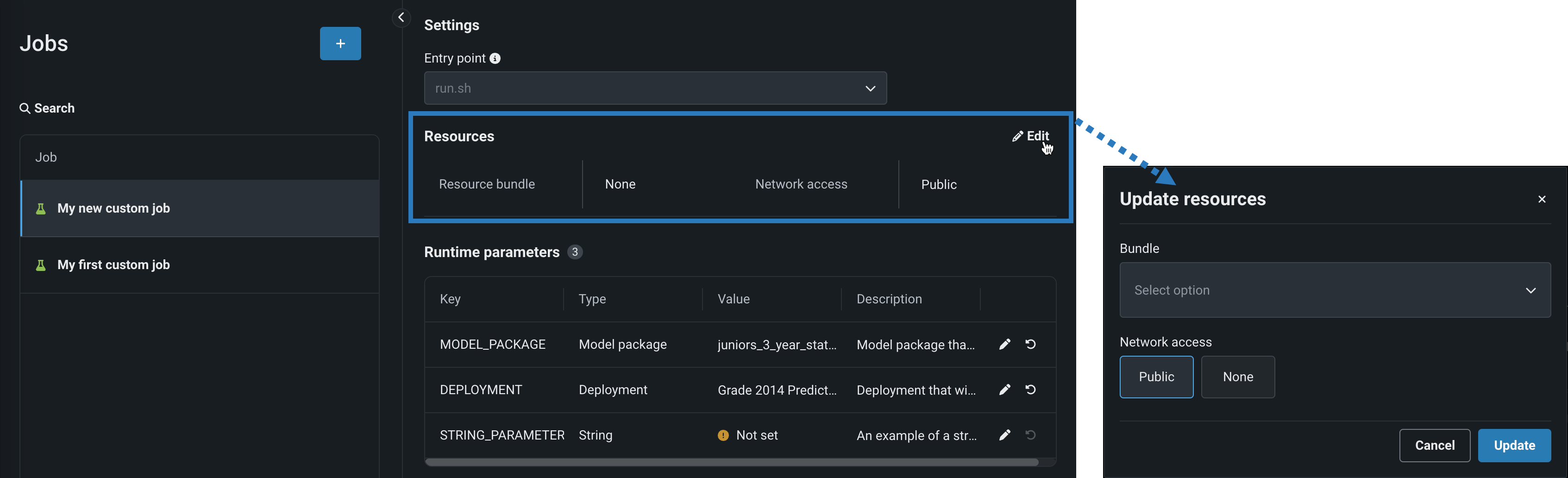
For more information, see the documentation.
Premium features¶
Automated deployment and replacement of Scoring Code in AzureML¶
Create a DataRobot-managed AzureML prediction environment to deploy DataRobot Scoring Code in AzureML. With the Managed by DataRobot option enabled, the model deployed externally to AzureML has access to MLOps management, including automatic Scoring Code replacement. Once you've created an AzureML prediction environment, you can deploy a Scoring Code-enabled model to that environment from the Model Registry:
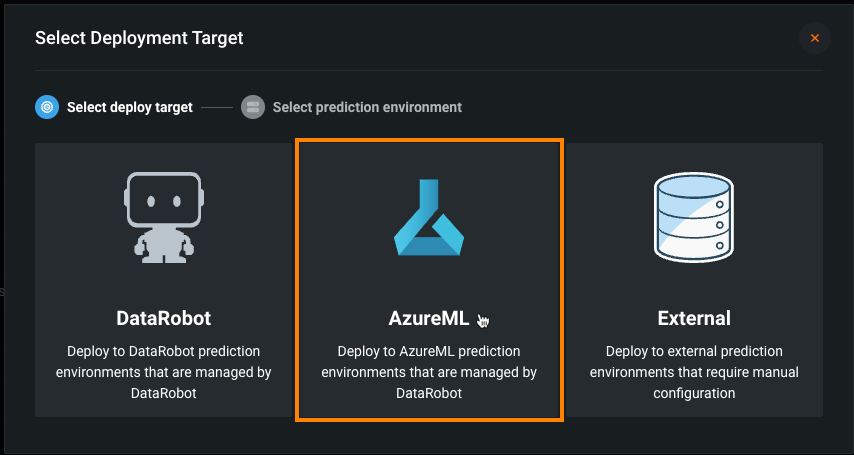
For more information, see the documentation.
Global models in the Registry¶
Deploy pre-trained, global models for predictive or generative use cases from the Registry (NextGen) and Model Registry (Classic). These high-quality, open-source models are trained and ready for deployment, allowing you to make predictions immediately after installing DataRobot. For GenAI use cases, you can find classifiers to identify prompt injection, toxicity, and sentiment, as well as a regressor to output a refusal score. Global models are available to all users; however, only administrators have edit rights. To identify global models on the Registry > Model directory page, locate the Global column and look for models with Yes:
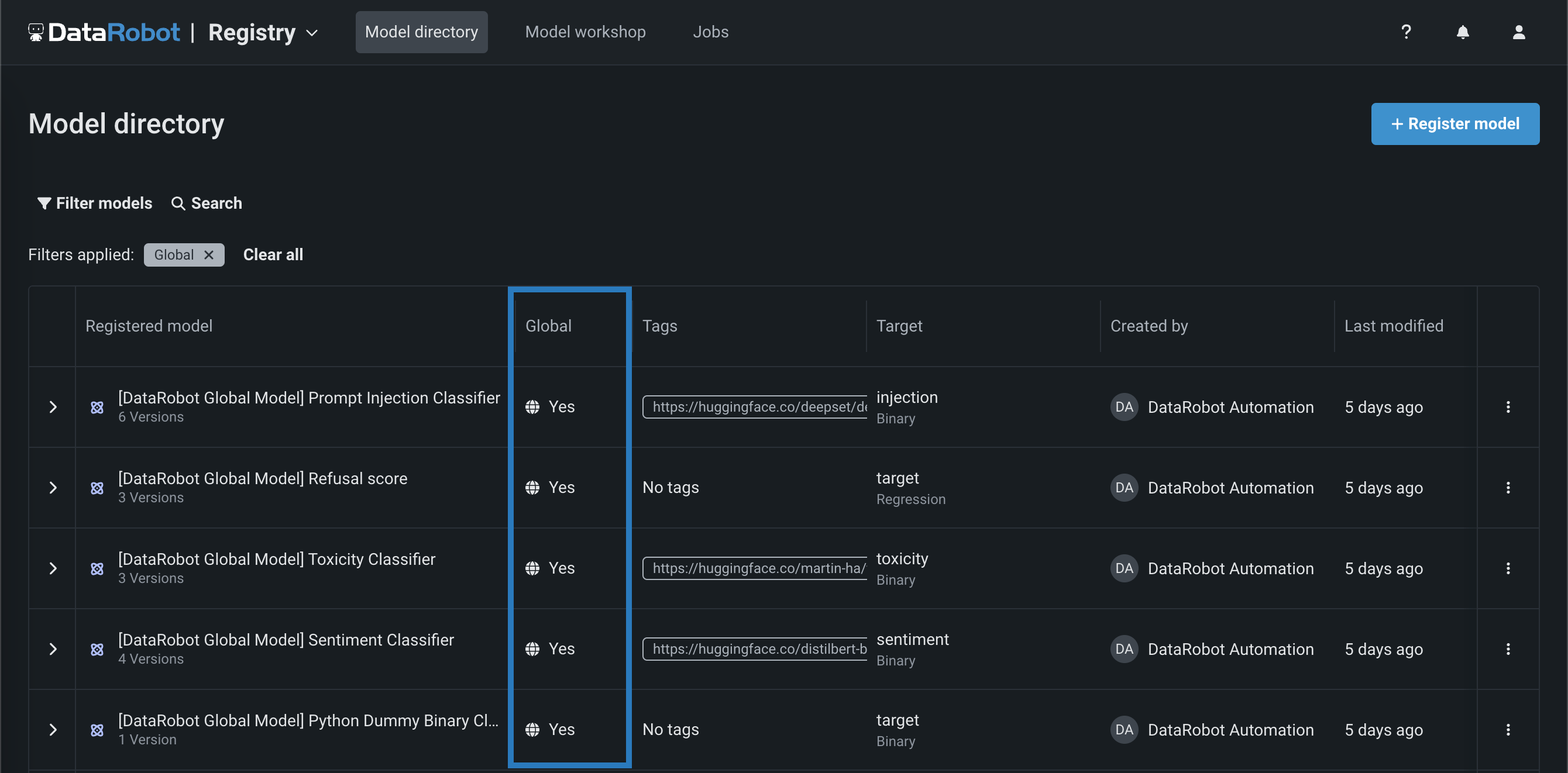
Build and use a chat generation Q&A application¶
Now available as a premium feature, you can create a chat generation Q&A application with DataRobot to explore knowledge base Q&A use cases while leveraging Generative AI to repeatedly make business decisions and showcase business value. The Q&A app offers an intuitive and responsive way to prototype, explore, and share the results of LLM models you've built. The Q&A app powers generative AI conversations backed by citations. Additionally, you can share the app with non-DataRobot users to expand its usability.
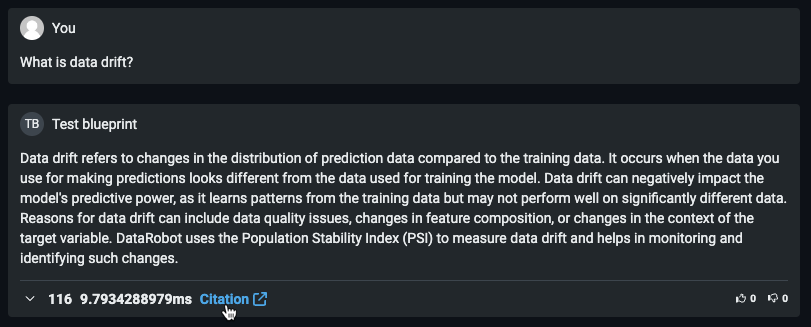
Premium documentation.
GA features¶
Notification policies for deployments¶
Configure deployment notifications through the creation of notification policies, you can configure and combine notification channels and templates. The notification template determines which events trigger a notification, and the channel determines which users are notified. The available notification channel types are webhook, email, Slack, Microsoft Teams, User, Group, and Custom Job. When you create a notification policy for a deployment, you can use a policy template without changes or as the basis of a new policy with modifications. You can also create an entirely new notification policy:
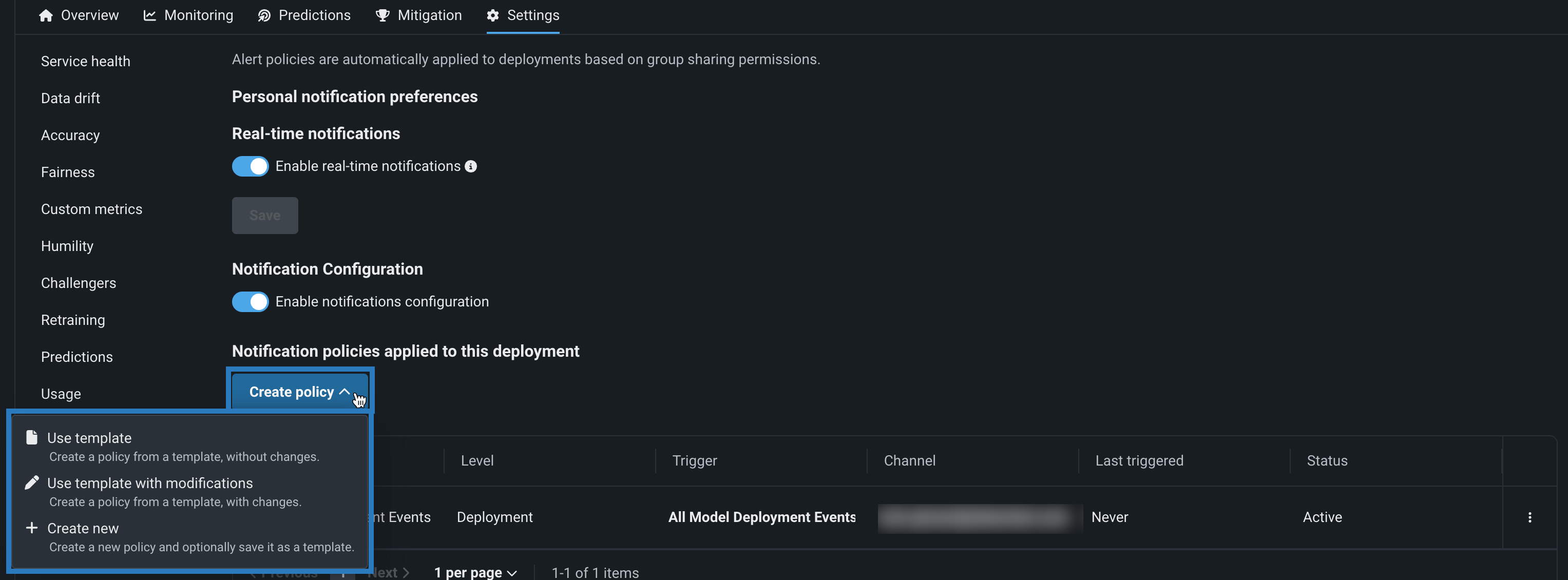
For more information, see the documentation.
Timeliness indicators for predictions and actuals¶
Deployments have several statuses to define their general health, including service health, data drift, and accuracy. These statuses are calculated based on the most recent available data. For deployments relying on batch predictions made in intervals greater than 24 hours, this method can result in an unknown status value on the prediction health indicators in the deployment inventory. Now generally available, deployment health indicators can retain the most recently calculated health status, presented along with timeliness status indicators to reveal when they are based on old data. You can determine the appropriate timeliness intervals for your deployments on a case-by-case basis. Once you've enabled timeliness tracking on a deployment's Usage > Settings tab, you can view timeliness indicators on the Usage tab and in the Deployments inventory:
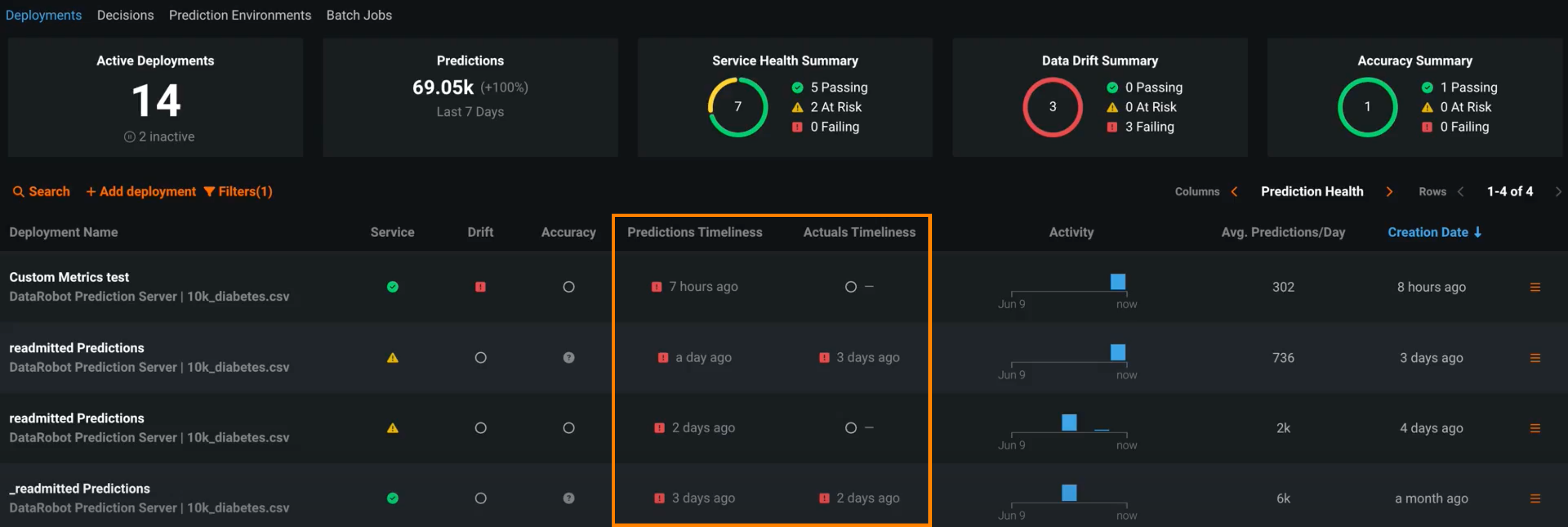
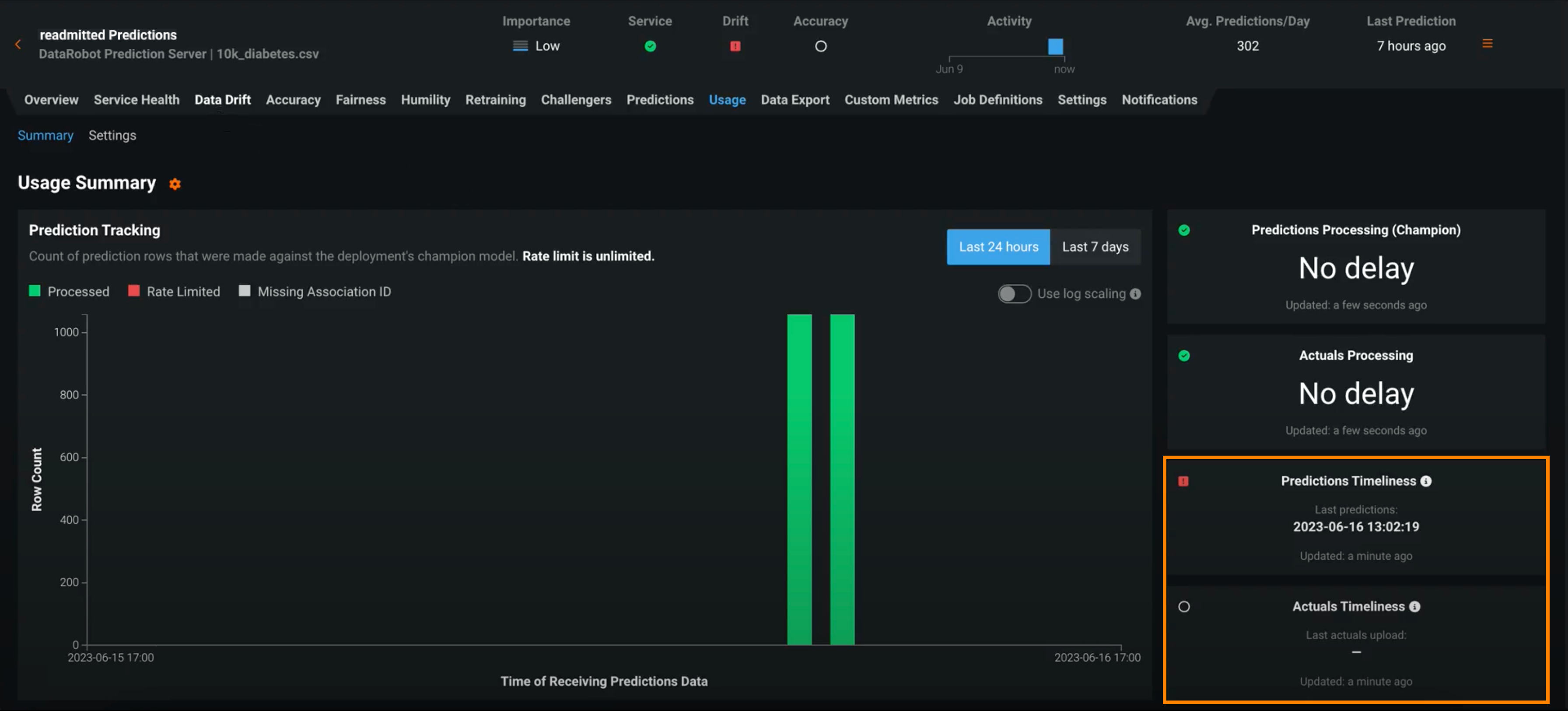
Custom apps hosting with DRApps CLI¶
DRApps is a simple command line interface (CLI) providing the tools required to host a custom application, such as a Streamlit app, in DataRobot using a DataRobot execution environment. This allows you to run apps without building your own Docker image. Custom applications don't provide any storage; however, you can access the full DataRobot API and other services. Alternatively, you can upload an AI App (Classic) or a custom application (NextGen) in a Docker container.
Paused custom applications
Custom applications are paused after a period of inactivity. The first time you access a paused custom application, a loading screen appears while it restarts.
For more information, see the documentation and the dr-apps repository.
Column name remapping for batch predictions¶
When configuring one-time or recurring batch predictions, you can change column names in the prediction job's output by mapping them to entries added in the Column names remapping section of the Prediction options. Click + Add column name remapping and define the Input column name to replace with the specified Output column name in the prediction output:

Tokenization improvements for Japanese text feature drift¶
Text tokenization for the Feature Details chart on the Data Drift tab is improved for Japanese text features, implementing word-gram-based data drift analysis with MeCab tokenization. In addition, default stop-word filtering is improved for Japanese text features.
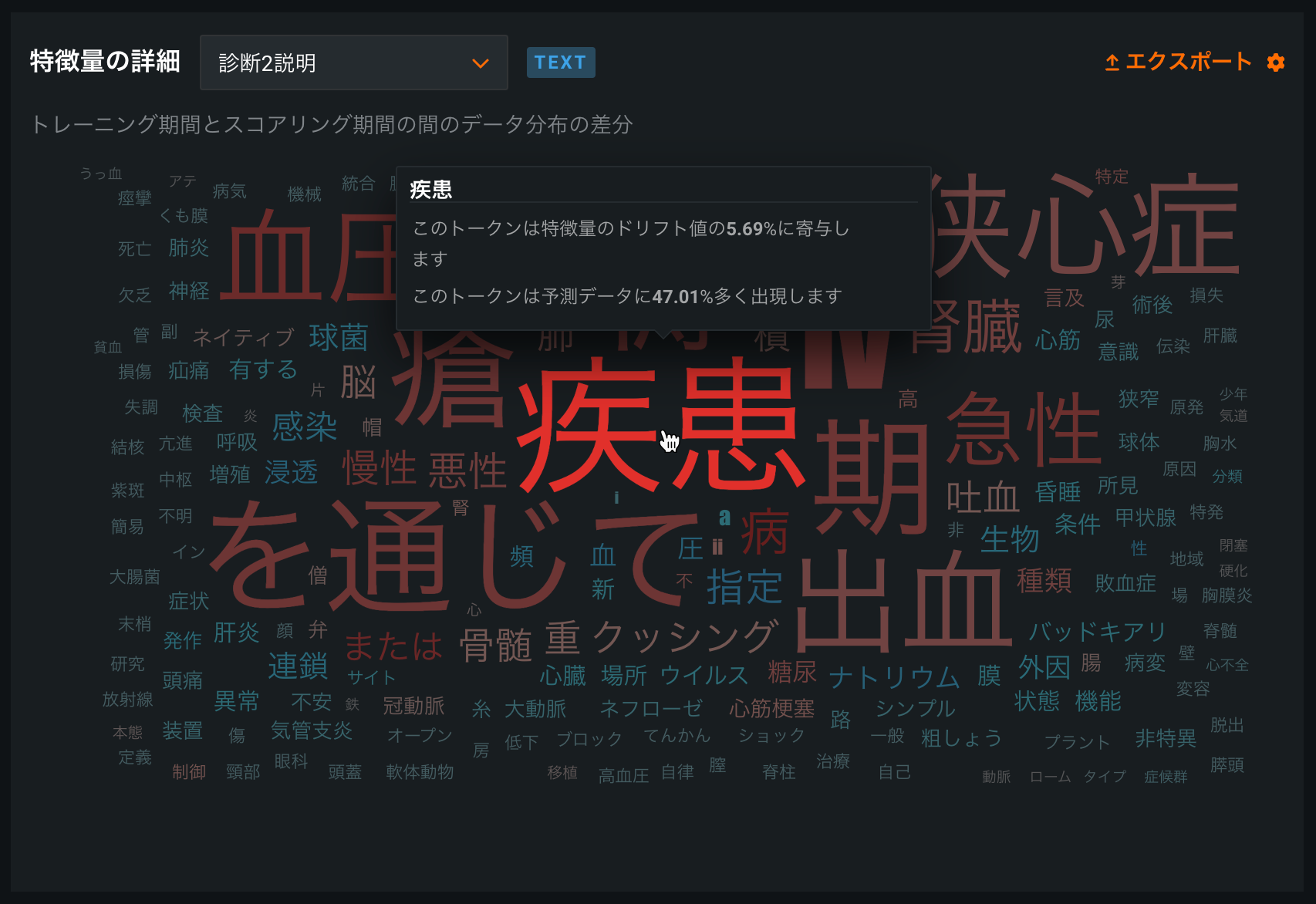
Batch monitoring for deployment predictions¶
View monitoring statistics organized by batch instead of by time with batch-enabled deployments. On the Predictions > Batch Management tab, you can create and manage batches. You can then add predictions to those batches and view service health, data drift, accuracy, and custom metric statistics by batch in your deployment. To create batches and assign predictions to a batch, you can use the UI or the API. In addition, each time a batch prediction or scheduled batch prediction job runs, a batch is created automatically, and every prediction from the job is added to that batch.

For more information, see the documentation.
Runtime parameters for custom models¶
Define runtime parameters through runtimeParameterDefinitions
in the model-metadata.yaml
file, and manage them on the Assemble tab of a custom model in the Runtime Parameters section:
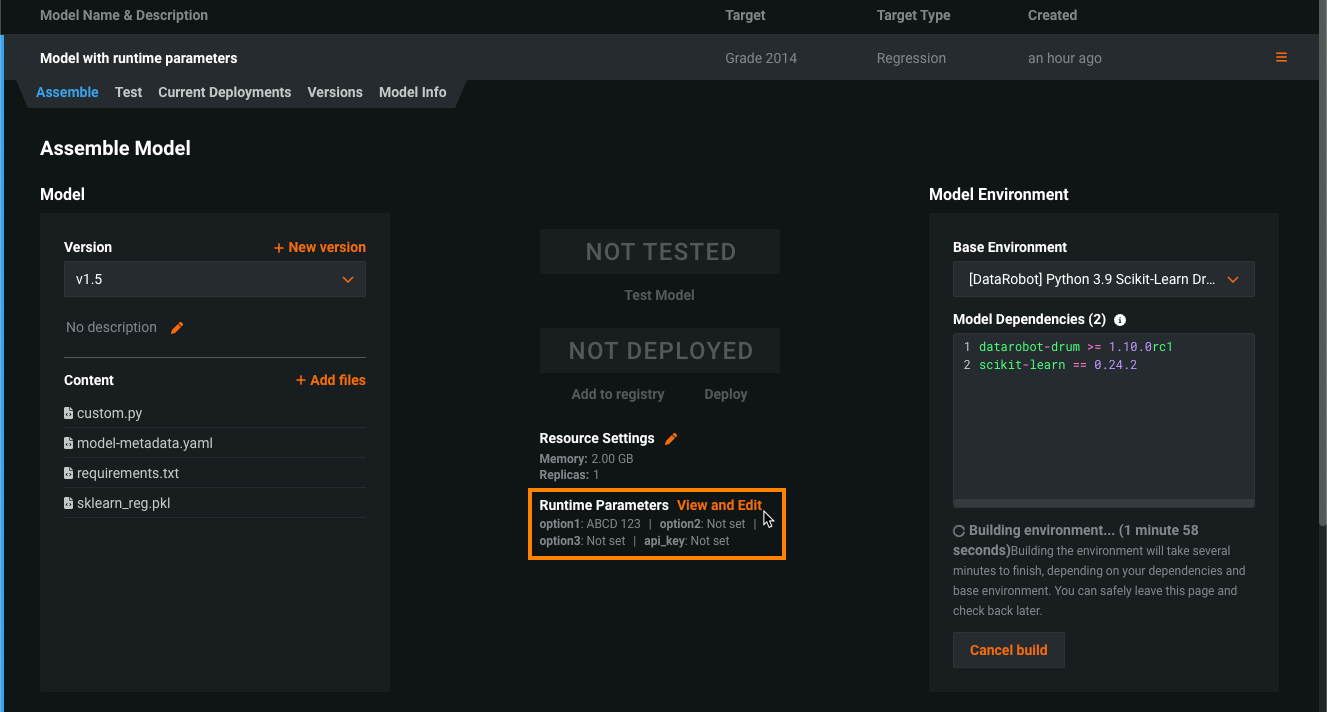
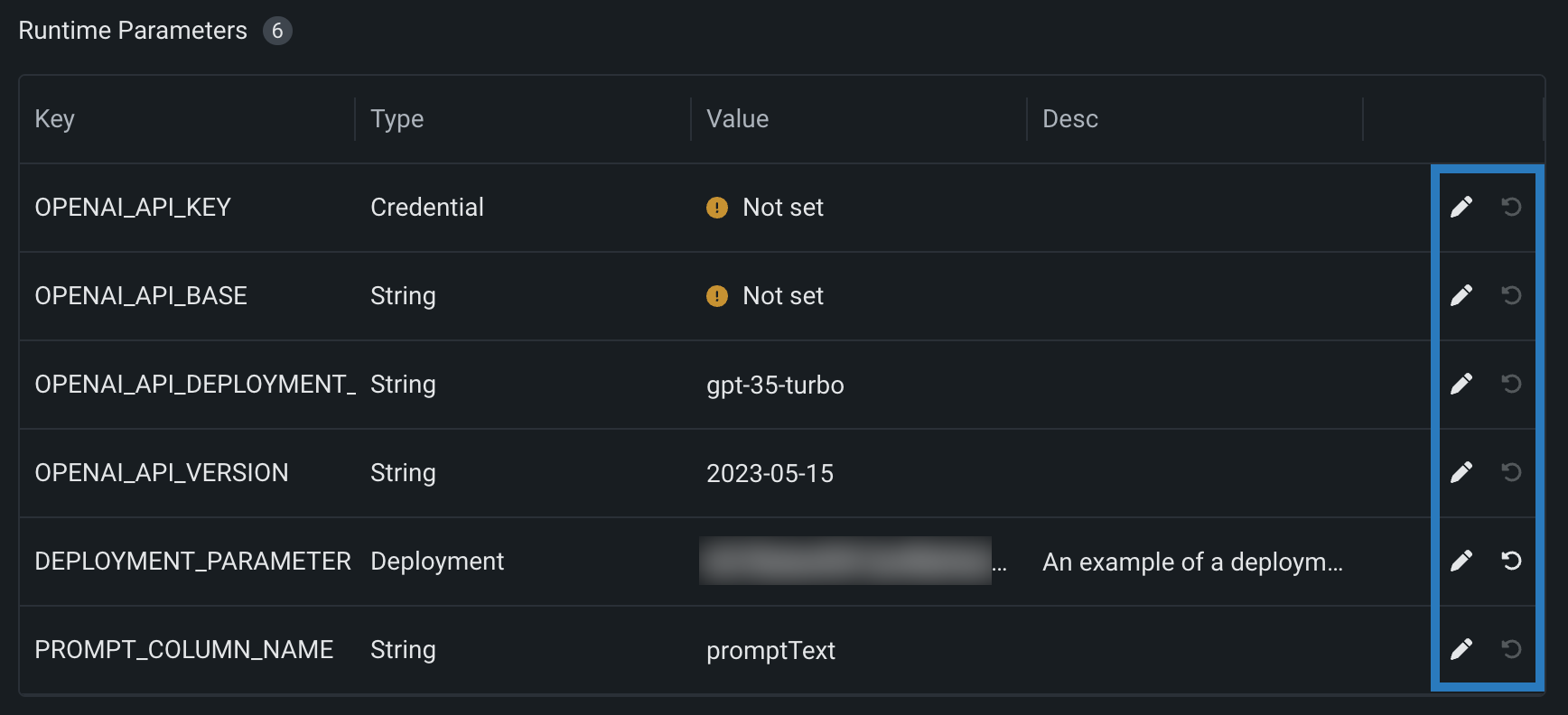
If any runtime parameters have allowEmpty: false
in the definition without a defaultValue
, you must set a value before registering the custom model.
For more information, see the Classic documentation or NextGen documentation.
New runtime parameter definition options for custom models¶
When you create runtime parameters for custom models through the model metadata, you can now set the type
key to boolean
or numeric
, in addition to string
or credential
. You can also add the following new, optional, runtimeParameterDefinitions
in model-metadata.yaml
:
Key | Description |
---|---|
defaultValue |
Set the default string value for the runtime parameter (the credential type doesn't support default values). |
minValue |
For numeric runtime parameters, set the minimum numeric value allowed in the runtime parameter. |
maxValue |
For numeric runtime parameters, set the maximum numeric value allowed in the runtime parameter. |
credentialType |
For credential runtime parameters, set the type of credentials the parameter should contain. |
allowEmpty |
Set the empty field policy for the runtime parameter:
|
For more information, see the documentation.
New environment variables for custom models¶
When you use a drop-in environment or a custom environment built on DRUM, your custom model code can reference several environment variables injected to facilitate access to the DataRobot client and MLOps connected client. The DATAROBOT_ENDPOINT
and DATAROBOT_API_TOKEN
environment variables require public network access, a premium feature available in NextGen and DataRobot Classic.
Environment Variable | Description |
---|---|
MLOPS_DEPLOYMENT_ID |
If a custom model is running in deployment mode (i.e., the custom model is deployed), the deployment ID is available. |
DATAROBOT_ENDPOINT |
If a custom model has public network access, the DataRobot endpoint URL is available. |
DATAROBOT_API_TOKEN |
If a custom model has public network access, your DataRobot API token is available. |
Custom jobs in the Model Registry¶
Create custom jobs in the Model Registry to implement automation (for example, custom tests) for your models and deployments. Each job serves as an automated workload, and the exit code determines if it passed or failed. You can run the custom jobs you create for one or more models or deployments. The automated workload you define when you assemble a custom job can make prediction requests, fetch inputs, and store outputs using DataRobot's Public API.

For more information, see the documentation.
Custom apps now generally available¶
Now generally available, you can create custom applications in DataRobot to share machine learning projects using web applications, including Streamlit, Dash, and R Shiny, from an image created in Docker. You can also use DRApps (a simple command line interface) to host a custom application in DataRobot using a DataRobot execution environment. This allows you to run apps without building your own Docker image. Custom applications don't provide any storage; however, you can access the full DataRobot API and other services. With this release, your custom applications are paused after a period of inactivity; the first time you access a paused custom application, a loading screen appears while it restarts.
Share custom applications¶
You can share custom apps with both DataRobot and non-DataRobot users, expanding the reach of custom applications you create. Access sharing functionality from the actions menu in the Apps workshop. To share a custom app with non-DataRobot users, you must toggle on Enable external sharing and specify the email domains and addresses that are permitted access to the app. DataRobot provides a link to share with these users after configuring the sharing settings.
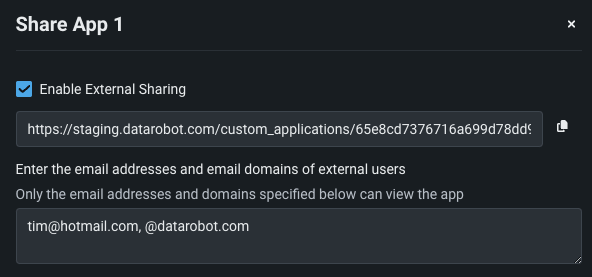
Preview features¶
Additional columns in custom model output¶
The score()
hook can return any number of extra columns, containing data of types string
, int
, float
, bool
, or datetime
. When additional columns are returned through the score()
method, the prediction response is as follows:
- For a tabular response (CSV), the additional columns are returned as part of the response table or dataframe.
- For a JSON response, the
extraModelOutput
key is returned alongside each row. This key is a dictionary containing the values of each additional column in the row.
Feature flag OFF by default: Enable Additional Custom Model Output in Prediction Responses
Preview documentation.
Disable column filtering for prediction requests¶
When you assemble a custom model, you can enable or disable column filtering for custom model predictions. The filtering setting you select is applied in the same way during custom model testing and deployment. By default, the target column is filtered out of prediction requests and, if training data is assigned, any additional columns not present in the training dataset are filtered out of any scoring requests sent to the model. Alternatively, if the prediction dataset is missing columns, an error message appears to notify you of the missing features.
You can disable this column filtering when you assemble a custom model. In the Model workshop, open a custom model to the Assemble tab, and, in the Settings section, under Column filtering, clear Exclude target column from requests (or, if training data is assigned, clear Exclude target and extra columns not in training data):
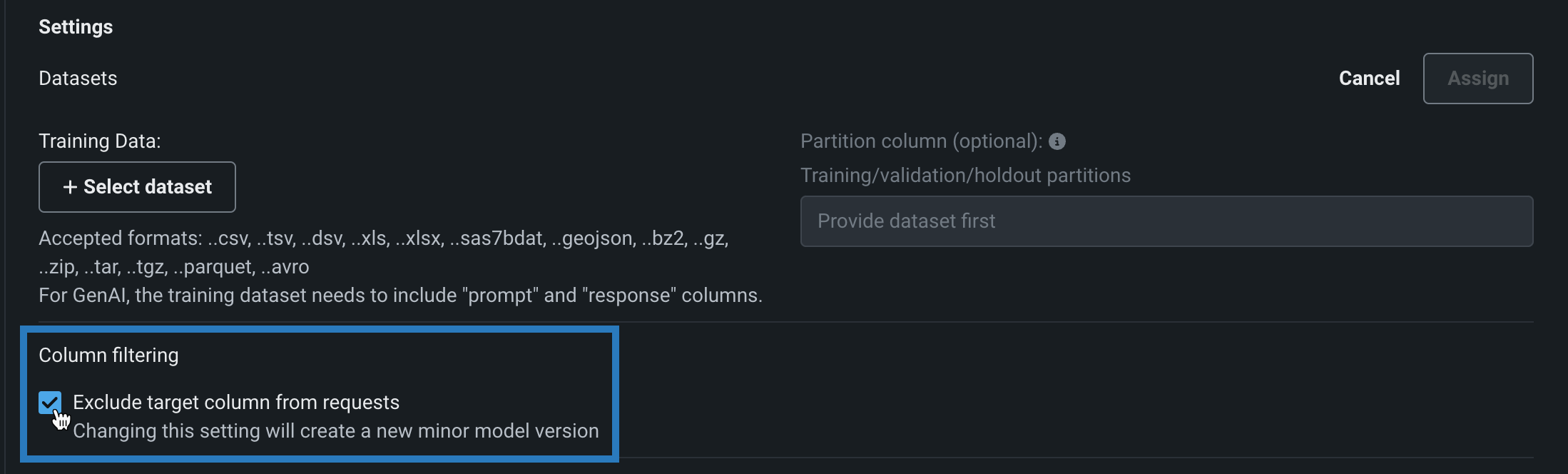
Feature flag OFF by default: Enable Feature Filtering for Custom Model Predictions
Preview documentation.
Monitoring agent in DataRobot¶
The monitoring agent typically runs outside of DataRobot, reporting metrics from a configured spooler populated by calls to the DataRobot MLOps library in the external model's code. Now available for preview, you can run the monitoring agent inside DataRobot by creating an external prediction environment with an external spooler's credentials and configuration details.
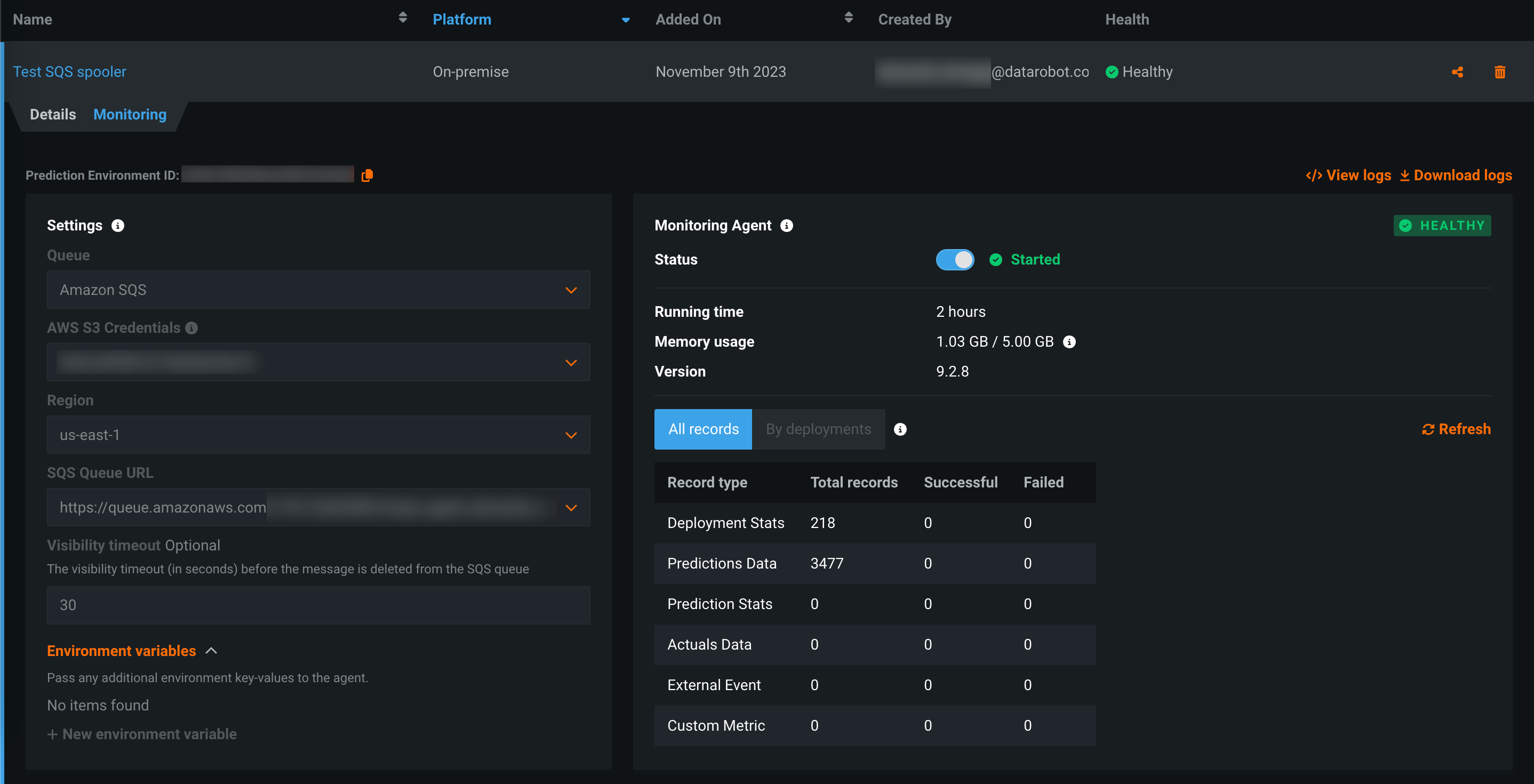
Preview documentation.
Feature flag ON by default: Monitoring Agent in DataRobot
Configure actuals and predictions upload limits¶
Now available as a preview feature, from the Usage tab, you can monitor the hourly, daily, and weekly upload limits configured for your organization's deployments. View charts that visualize the number of predictions and actuals processed and tiles that display the table size limits for returned prediction results.
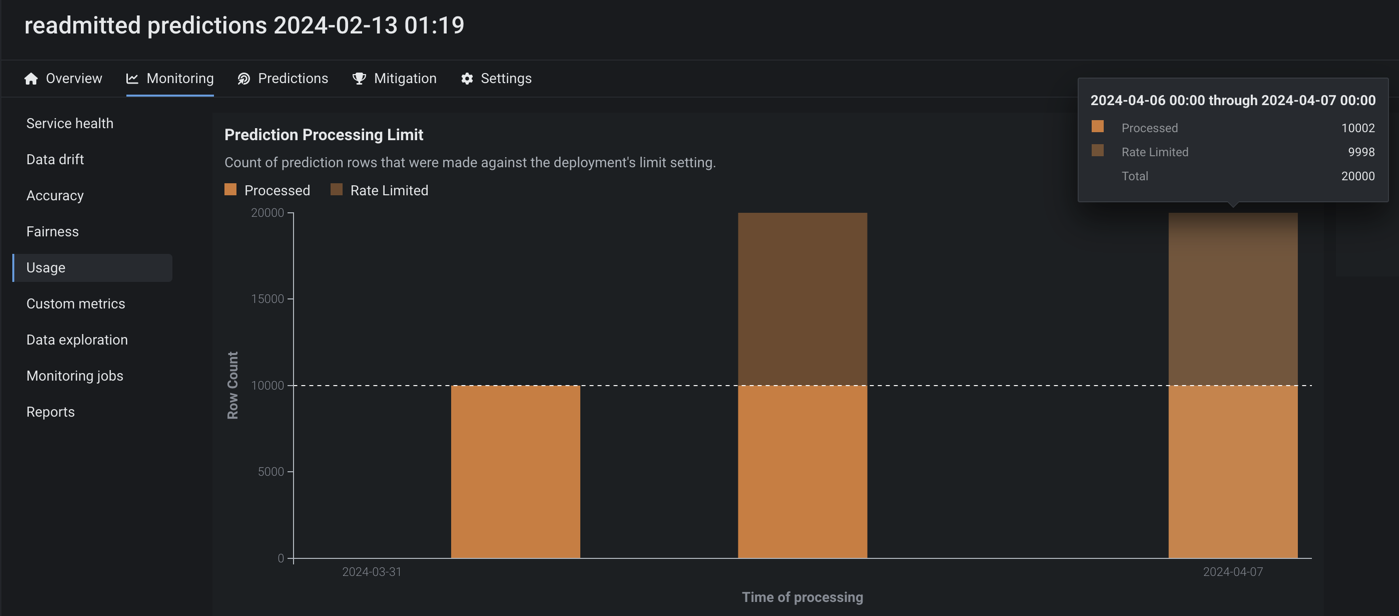
Feature flag OFF by default: Enable Configurable Prediction and Actuals Limits
Preview documentation.
Configure runtime parameters and resource bundles for custom applications¶
Now available as a preview feature, you can configure the resources and runtime parameters for application sources in the NextGen Registry. The resources bundle determines the maximum amount of memory and CPU that an application can consume to minimize potential environment errors in production. You can create and define runtime parameters used by the custom application by including them in the metadata.yaml
file built from the application source.
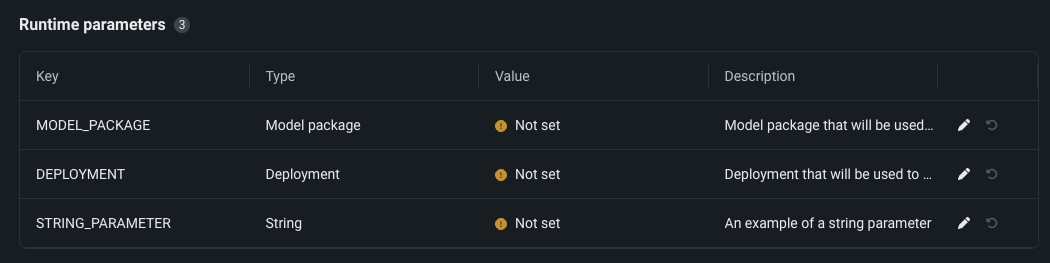
Feature flags OFF by default: Enable Runtime Parameters and Resource Limits, Enable Resource Bundles
Preview documentation.
Resource bundles for custom models¶
Select a Resource bundle—instead of Memory—when you assemble a model and configure the resource settings. Resource bundles allow you to choose from various CPU and GPU hardware platforms for building and testing custom models. In a custom model's Settings section, open the Resources settings to select a resource bundle. In this example, the model is built to be tested and deployed on an NVIDIA A10 device.
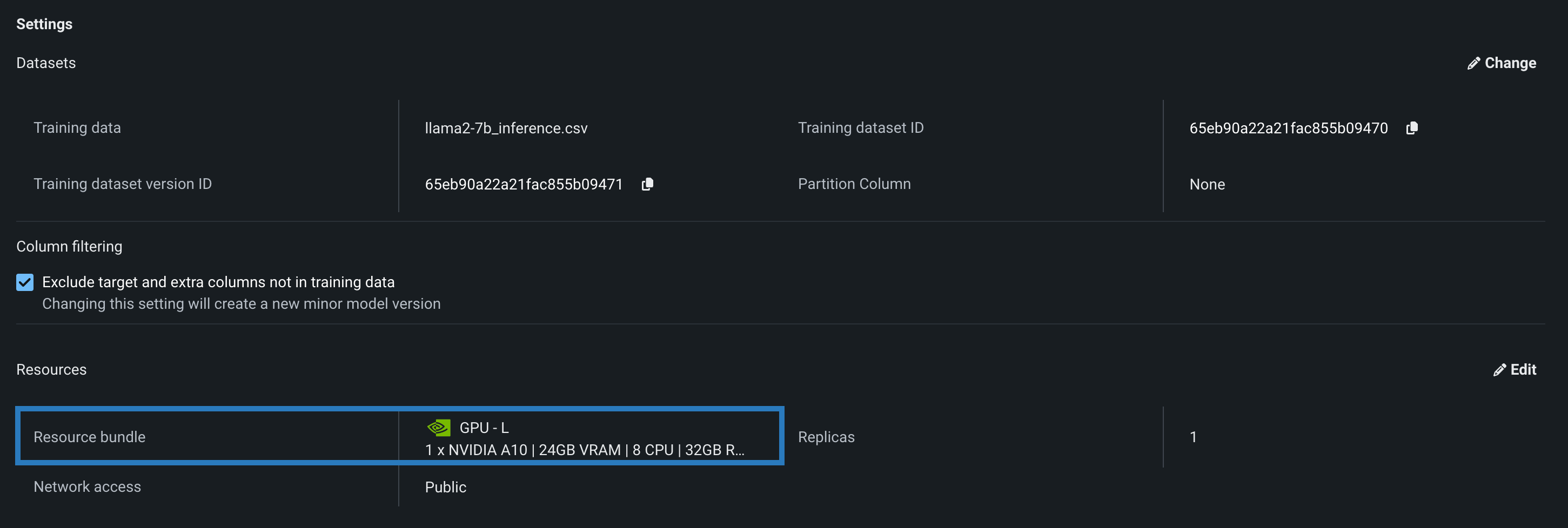
Click Edit to open the Update resource settings dialog box and, in the resource Bundle field, review the CPU and NVIDIA GPU devices available as build environments:
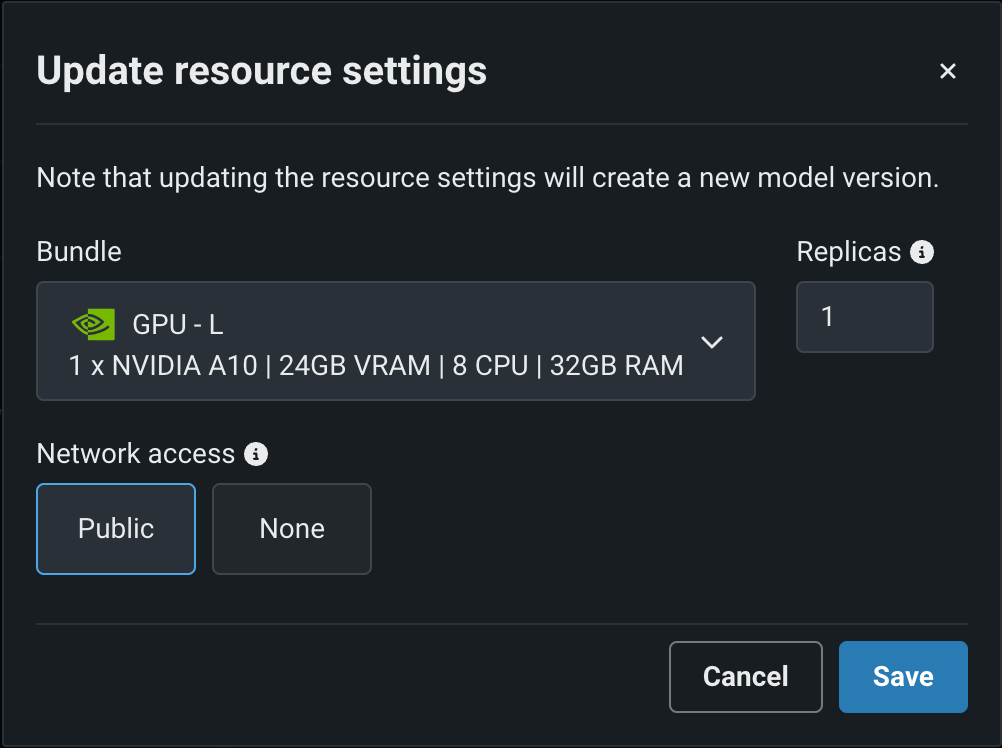
Feature flags OFF by default: Enable Resource Bundles, Enable Custom Model GPU Inference
Preview documentation.
Evaluation and moderation for text generation models¶
Evaluation and moderation guardrails help your organization block prompt injection and hateful, toxic, or inappropriate prompts and responses. It can also prevent hallucinations or low-confidence responses and, more generally, keep the model on topic. In addition, these guardrails can safeguard against the sharing of personally identifiable information (PII). Many evaluation and moderation guardrails connect a deployed text generation model (LLM) to a deployed guard model. These guard models make predictions on LLM prompts and responses and then report these predictions and statistics to the central LLM deployment. To use evaluation and moderation guardrails, first, create and deploy guard models to make predictions on an LLM's prompts or responses; for example, a guard model could identify prompt injection or toxic responses. Then, when you create a custom model with the Text Generation target type, define one or more evaluation and moderation guardrails.
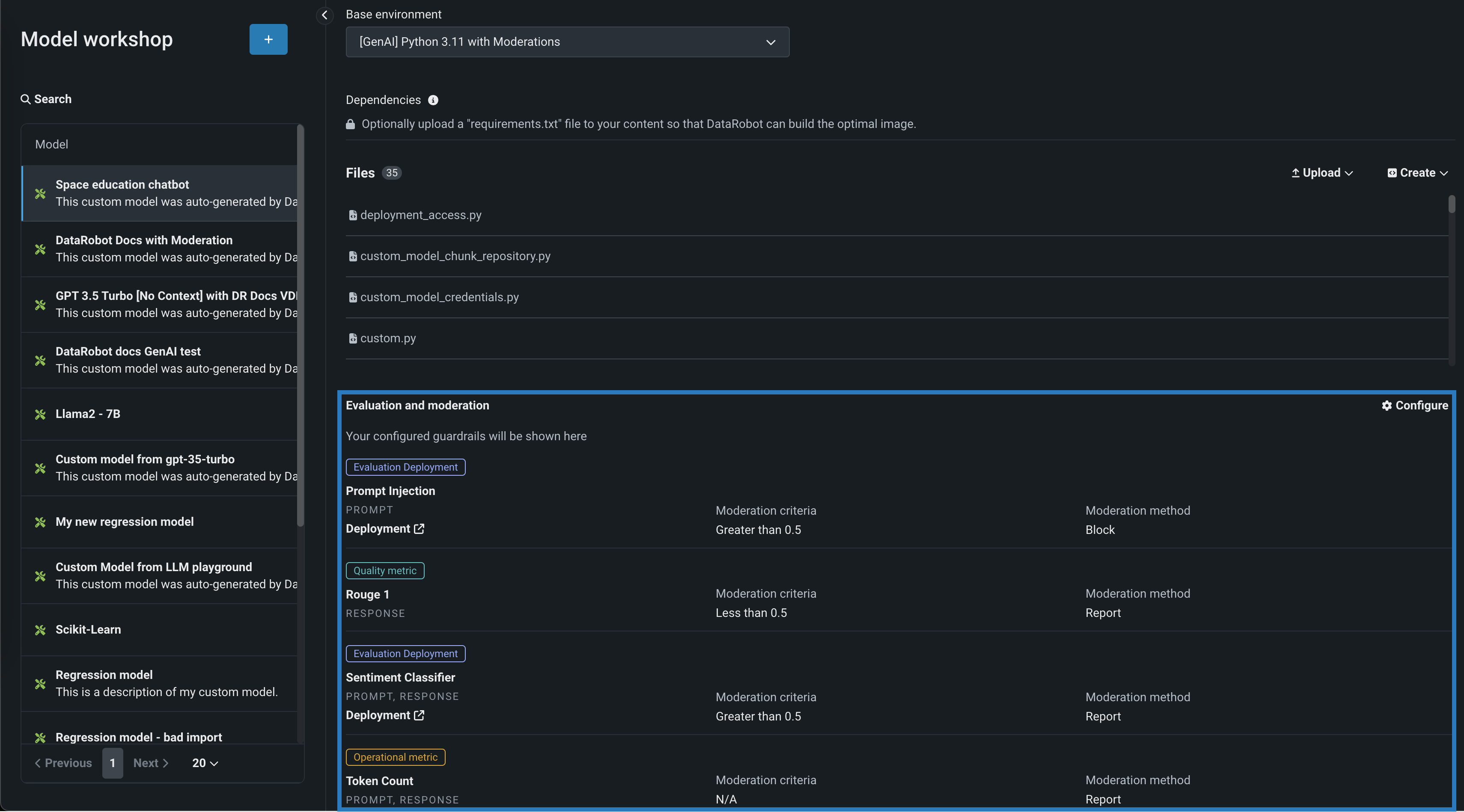
Feature flags OFF by default: Enable Moderation Guardrails, Enable Global Models in the Model Registry (Premium), Enable Additional Custom Model Output in Prediction Responses
Preview documentation.
Generative AI with NeMo Guardrails on NVIDIA GPUs¶
Use NVIDIA with DataRobot to quickly build out end-to-end generative AI (GenAI) capabilities by unlocking accelerated performance and leveraging the very best of open-source models and guardrails. The DataRobot integration with NVIDIA creates an inference software stack that provides full, end-to-end Generative AI capability, ensuring performance, governance, and safety through significant functionality out of the box.
When you create a custom Generative AI model in the NextGen DataRobot Model workshop, you can select an [NVIDIA] Triton Inference Server (vLLM backend) base environment. DataRobot has natively built in the NVIDIA Triton Inference Server to provide extra acceleration for all of your GPU-based models as you build and deploy them onto NVIDIA devices.
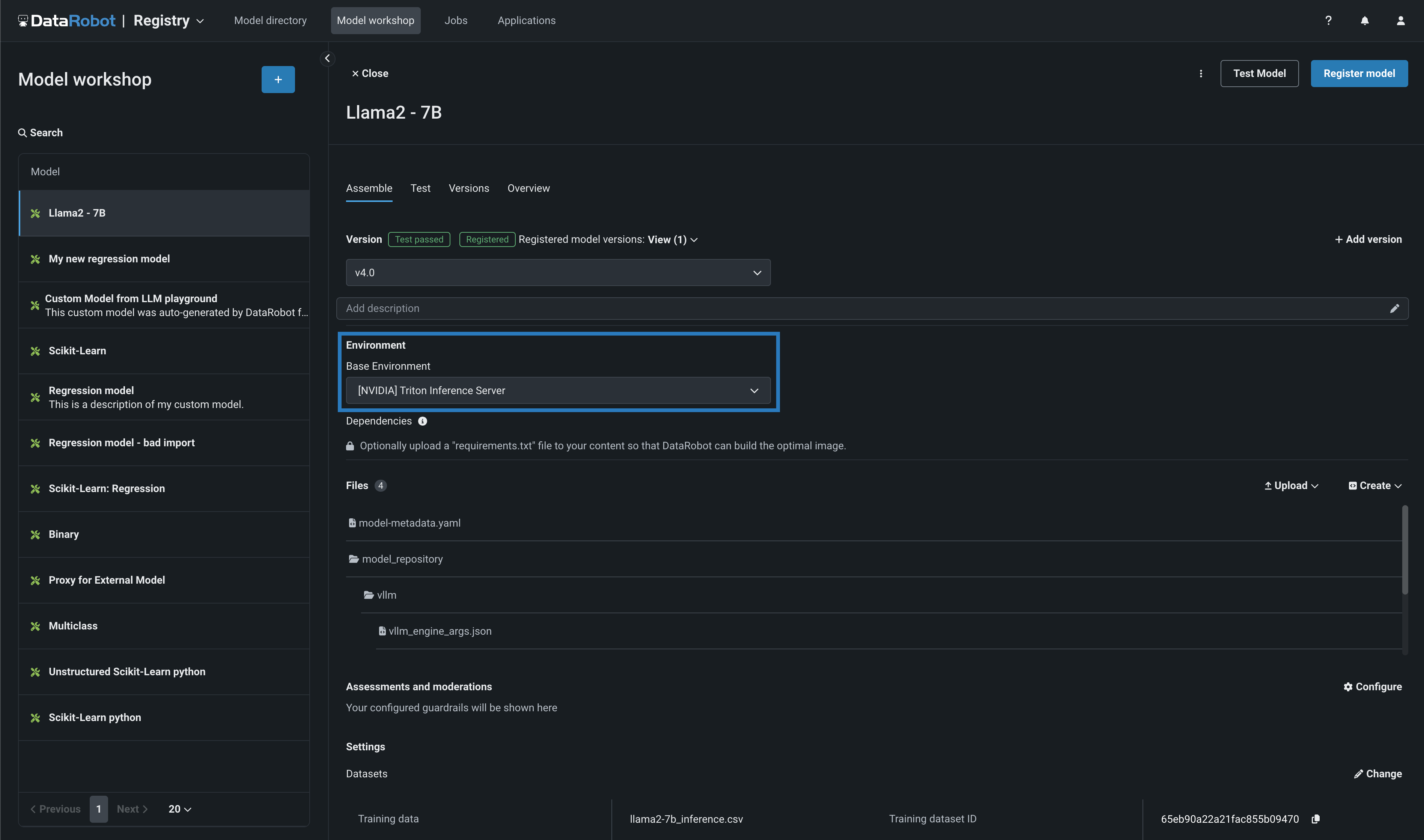
Then, navigating to the custom model's resources settings, you can select a resource bundle from the range of NVIDIA devices available as build environments in DataRobot.
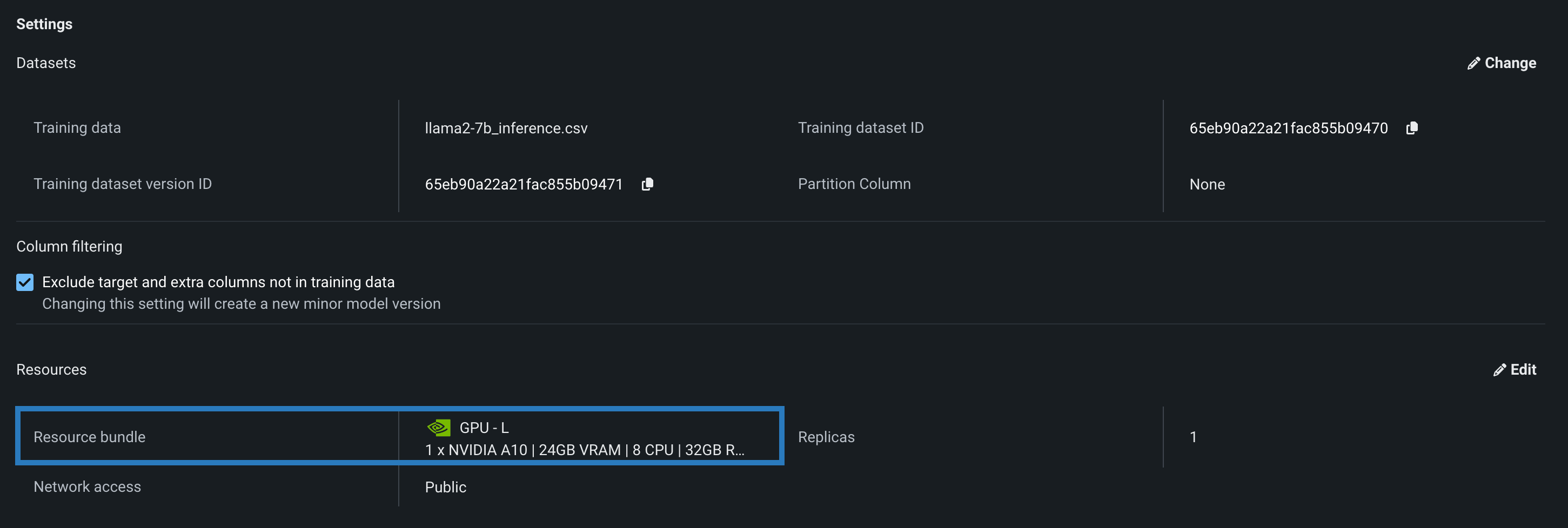
In addition, DataRobot also provides a powerful interface to create custom metrics through an integration with NeMo Guardrails. The integration with NeMo provides powerful rails to ensure your model stays on topic, using interventions to block prompts and completions if they violate the "on topic" principles provided by NeMo.
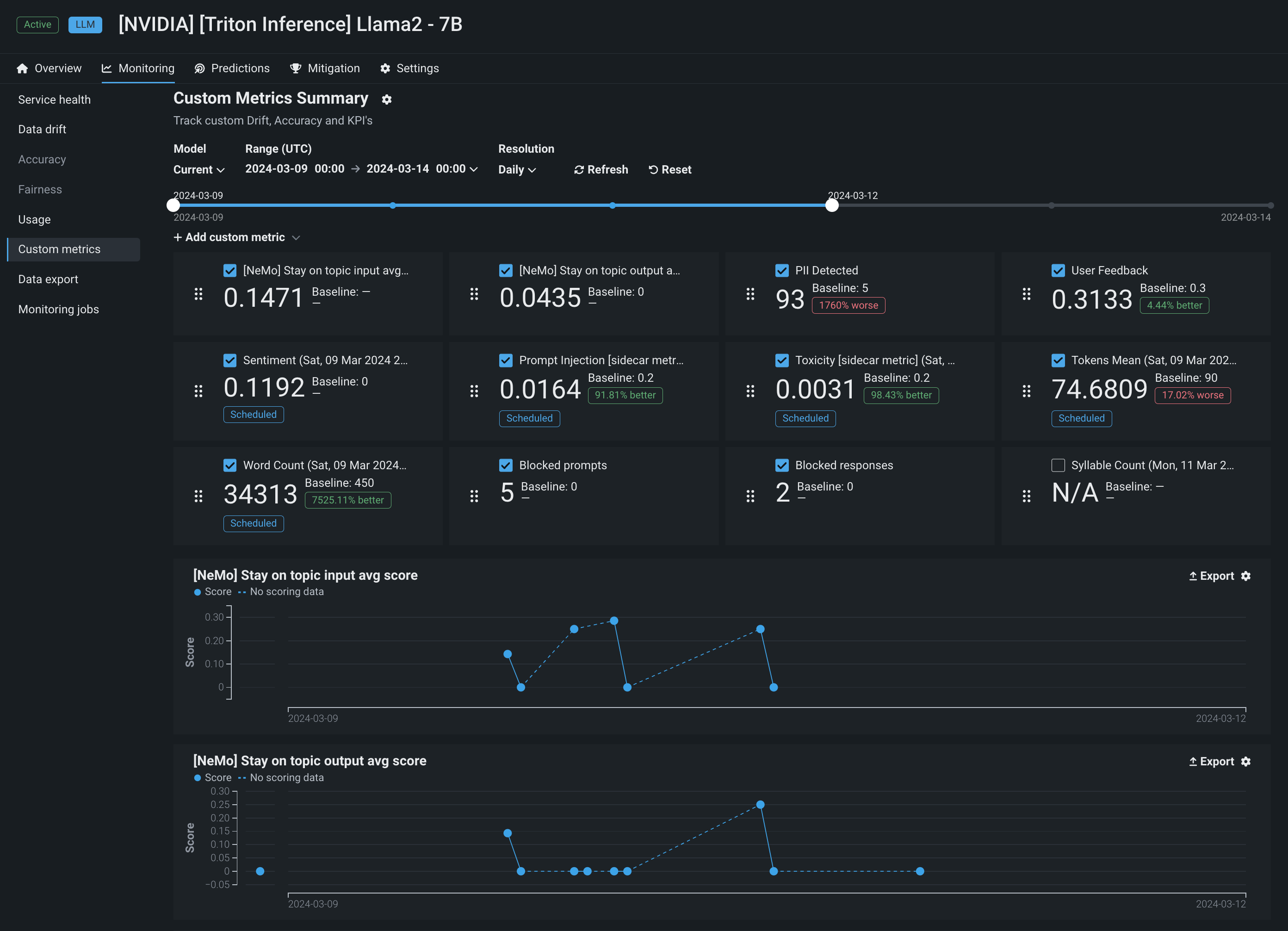
Time series custom models¶
Create time series custom models by selecting Time Series (Binary) or Time Series (Regression) as a Target type and configuring time series-specific fields, in addition to the fields required for binary classification and regression models:
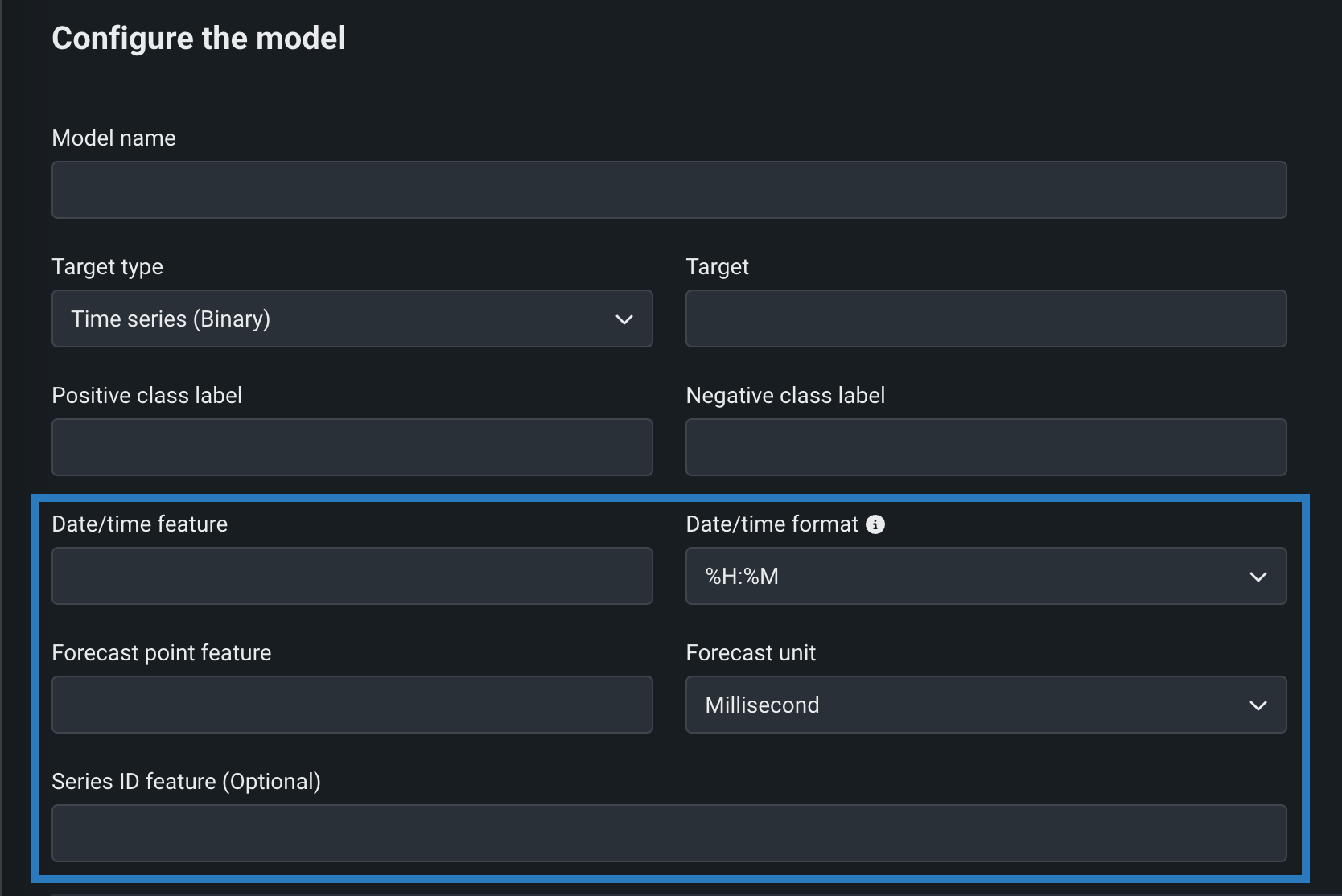
Feature flags OFF by default: Enable Time Series Custom Models, Enable Feature Filtering for Custom Model Predictions
Preview documentation.
Data quality analysis for deployments¶
On the Data exploration tab of a Generative AI deployment, click Data quality to explore prompts, responses, user ratings, and custom metrics matched by association ID. This view provides insight into the quality of the Generative AI model's responses, as rated by users and based on any Generative AI custom metrics you implement:
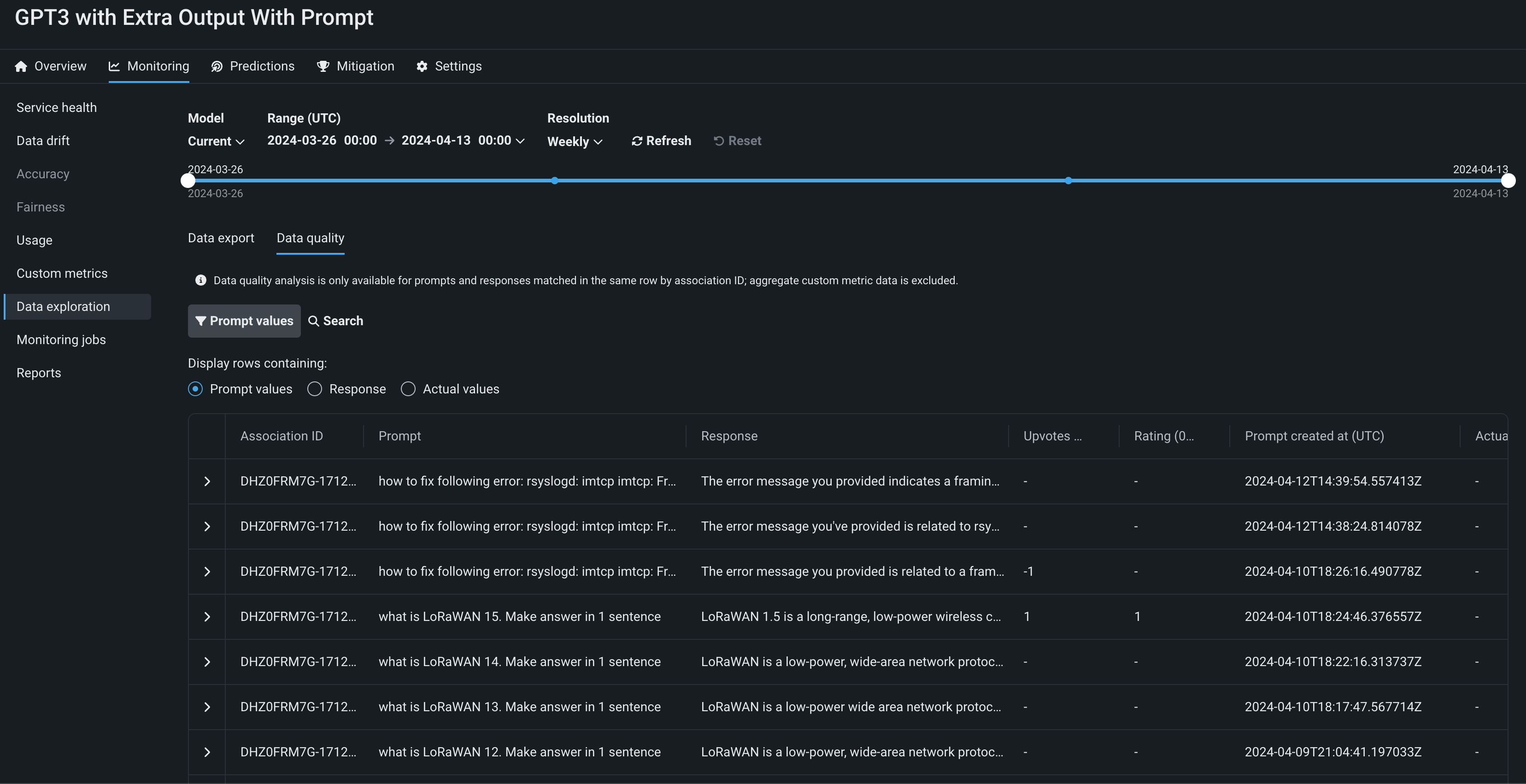
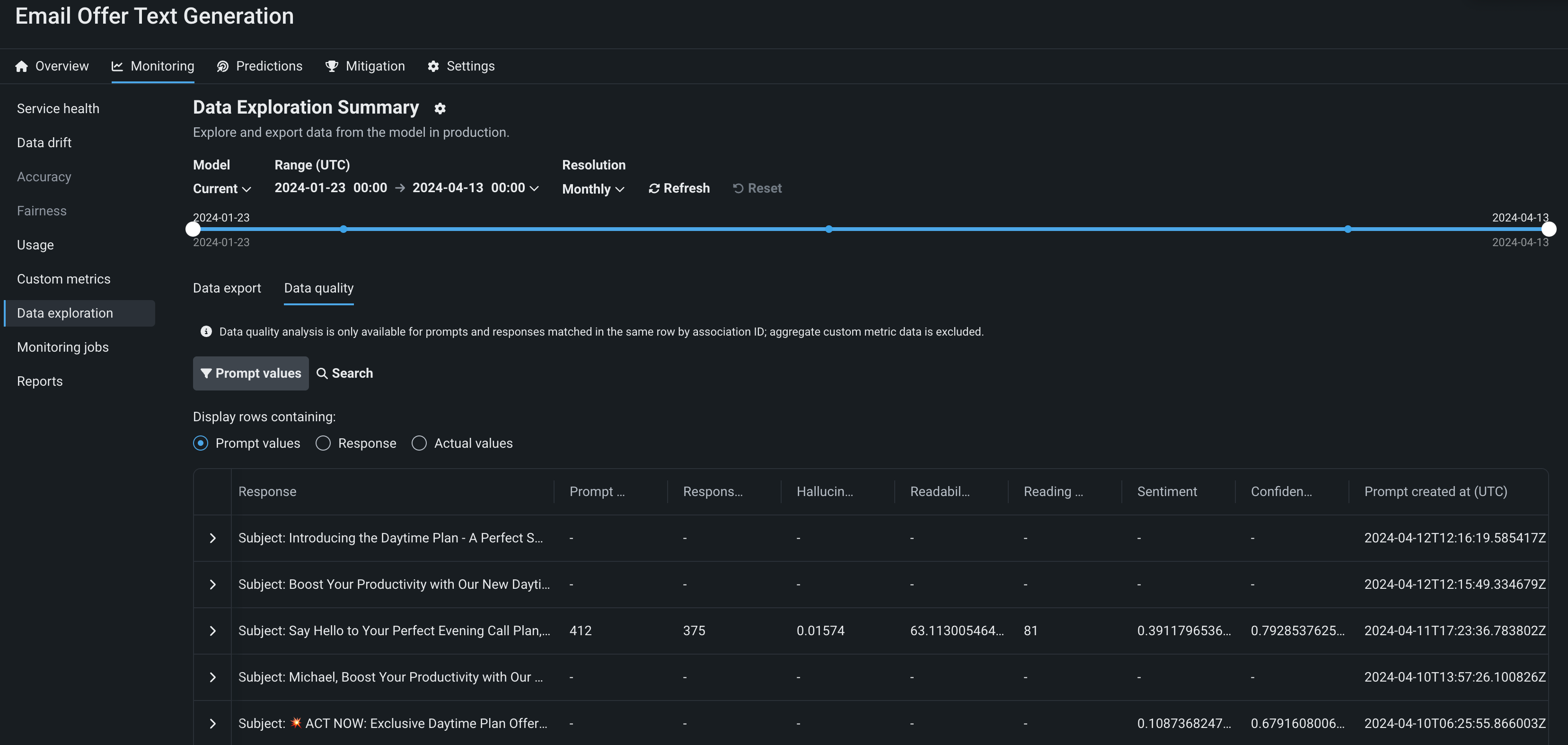
Feature flags OFF by default: Enable Data Quality Table for Text Generation Target Types (Premium feature), Enable Actuals Storage for Generative Models (Premium feature)
Preview documentation.
Custom metric and retraining jobs¶
When you create a custom job on the NextGen Registry > Jobs page, you can now create custom metric and retraining jobs. Click + Add new (or the button when the custom job panel is open):
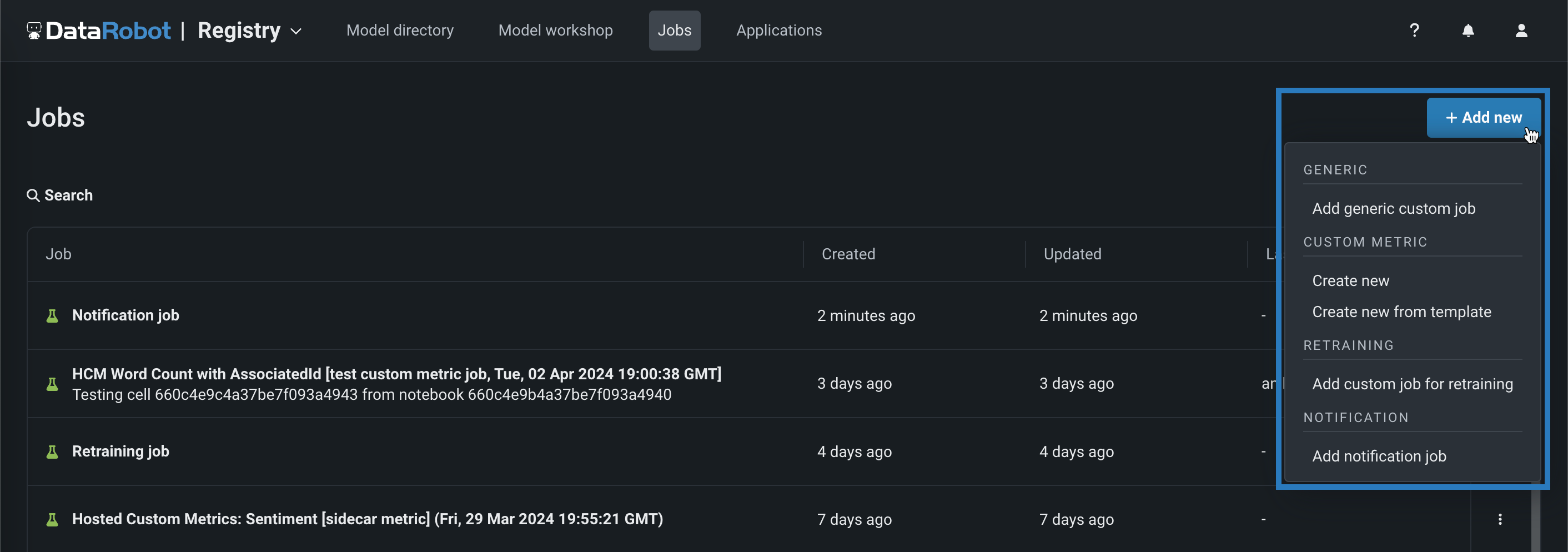
Custom job type | Description |
---|---|
Custom Metric | |
Create new | Add a custom job for a new hosted custom metric, defining the custom metric settings and associating the metric with a deployment. |
Create new from template | Add a custom job for a custom metric from a template provided by DataRobot, associating the metric with a deployment and setting a baseline. |
Retraining | |
Add custom job for retraining | Add a custom job implementing a code-based retraining policy. After you create a custom job for retraining, you can add it to a deployment as a retraining policy. |
When you create a custom metric job, you can connect the job to a deployment from the Assemble tab. In the Connected deployments panel, click + Connect to deployment. Then, you can edit the Custom metric name and select a Deployment ID, set a Baseline, and click Connect.
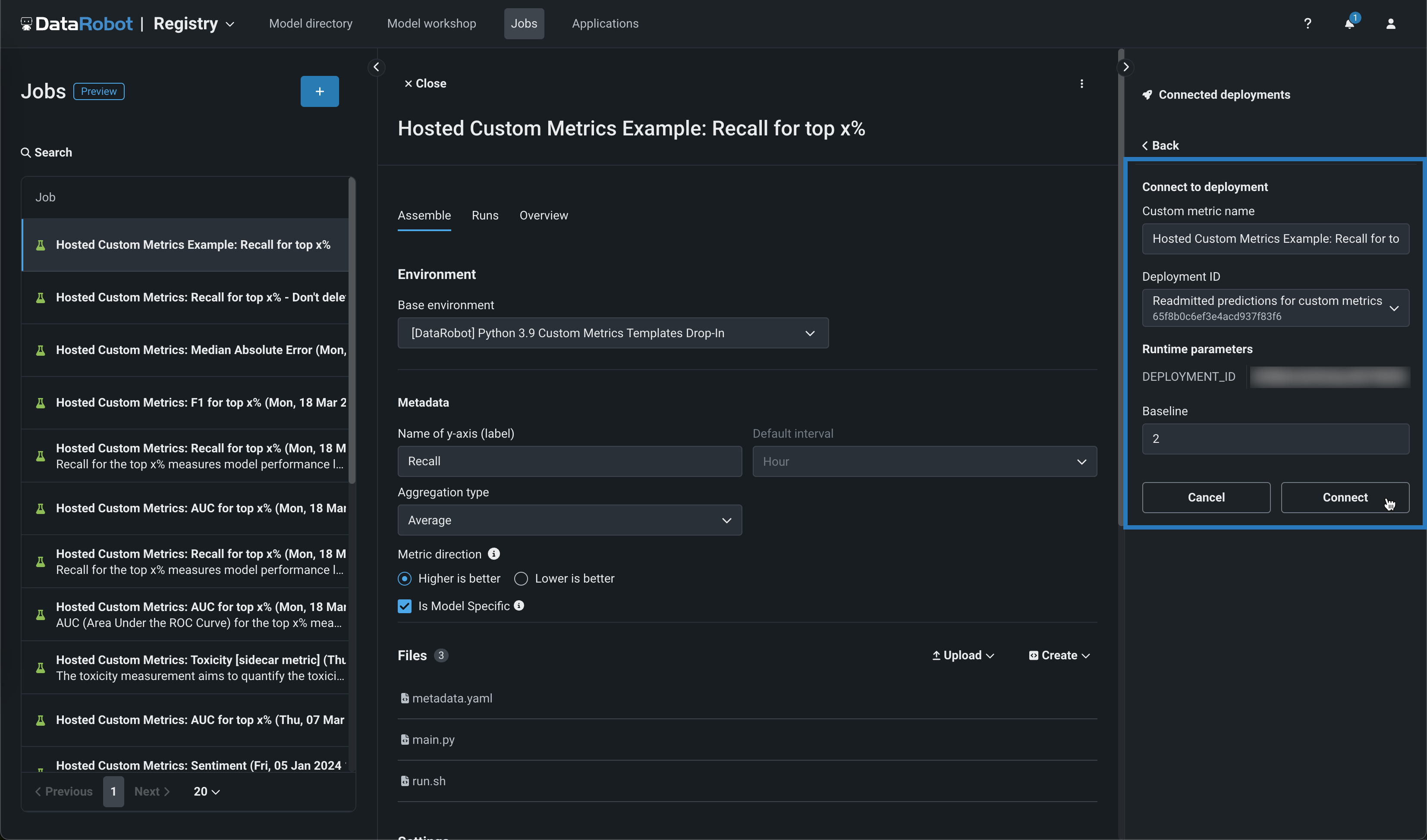
Feature flags OFF by default for custom metric jobs: Enable Hosted Custom Metrics, Enable Custom Jobs, Enable Notebooks Custom Environments
Feature flags OFF by default for retraining jobs: Enable Custom Job Based Retraining Polices, Enable Custom Jobs, Enable Notebooks Custom Environments
Preview documentation.
Wrangler recipes in batch predictions¶
Use a deployment's Predictions > Make predictions tab to efficiently score Wrangler datasets with a deployed model by making batch predictions. Batch predictions are a method of making predictions with large datasets, in which you pass input data and get predictions for each row. In the Prediction dataset box, click Choose file > Wrangler to make predictions with a Wrangler dataset:
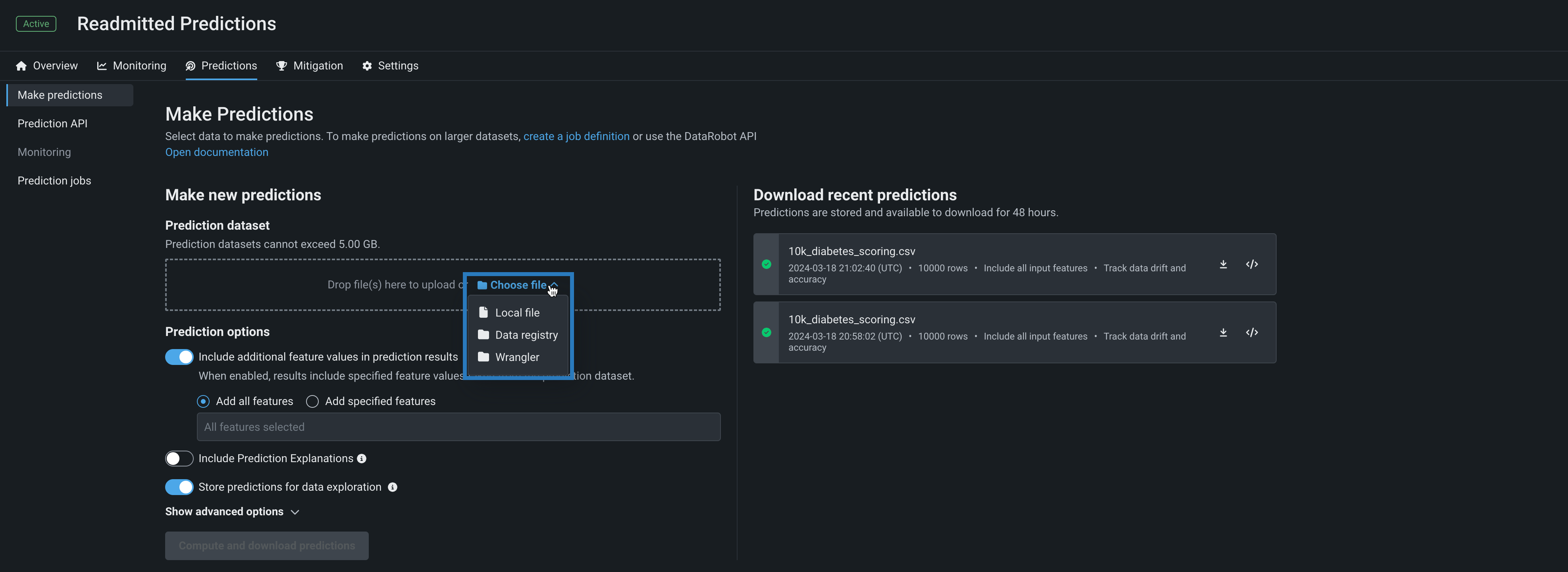
Predictions in Workbench
Wrangler is also available as a prediction dataset source in Workbench. To make predictions with a model before deployment, select the model from the Models list in an experiment and then click Model actions > Make predictions.
You can also schedule batch prediction jobs by specifying the prediction data source and destination and determining when DataRobot runs the predictions.
Feature flags OFF by default: Enable Wrangler Recipes for Batch Prediction Jobs, Enable Recipe Management in Workbench
Preview documentation.
Manage custom applications in the Registry¶
Now available for preview, the Applications page in the NextGen Registry is home to all built custom applications and application sources available to you. You can now create application sources, which contain the files, environment, and runtime parameters for custom applications you want to build. You can now build custom applications directly from these sources. You can also use the Applications page to manage applications by sharing or deleting them.
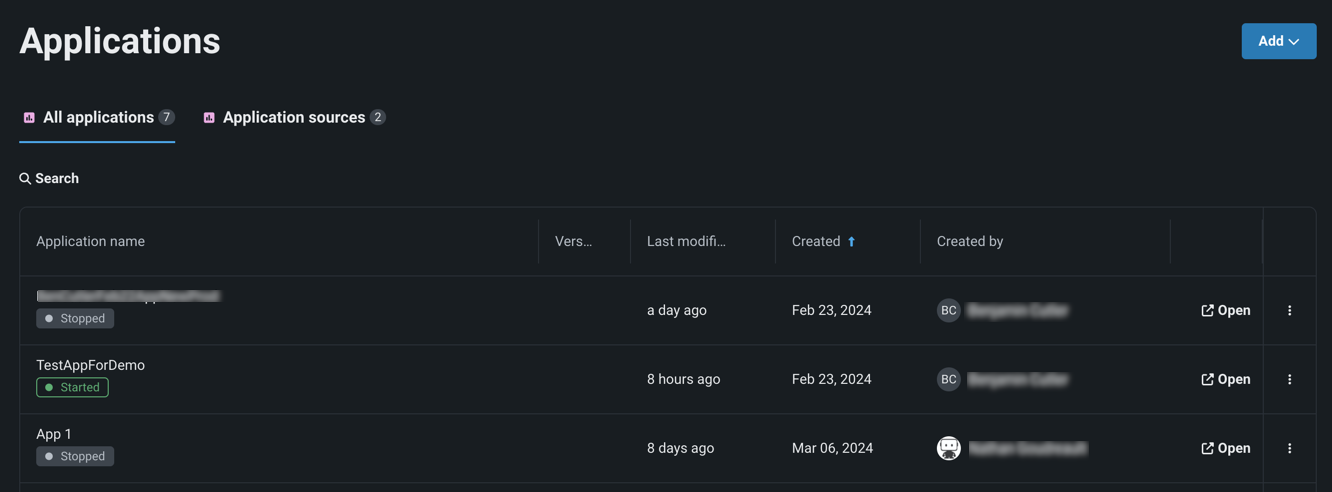
Preview documentation.
Feature flag OFF by default: Enable Custom Applications Workshop
Deprecations and migrations¶
Custom model training data assignment update¶
In DataRobot version 10.1, the assignment of training data to a custom model version—announced in the DataRobot version 9.1 release—replaces the deprecated method of assigning training data at the custom model level. This means that the “per custom model version” method becomes the default, and the “per custom model” method is removed. In preparation, you can review the manual conversion instructions in the Custom model training data assignment update documentation:
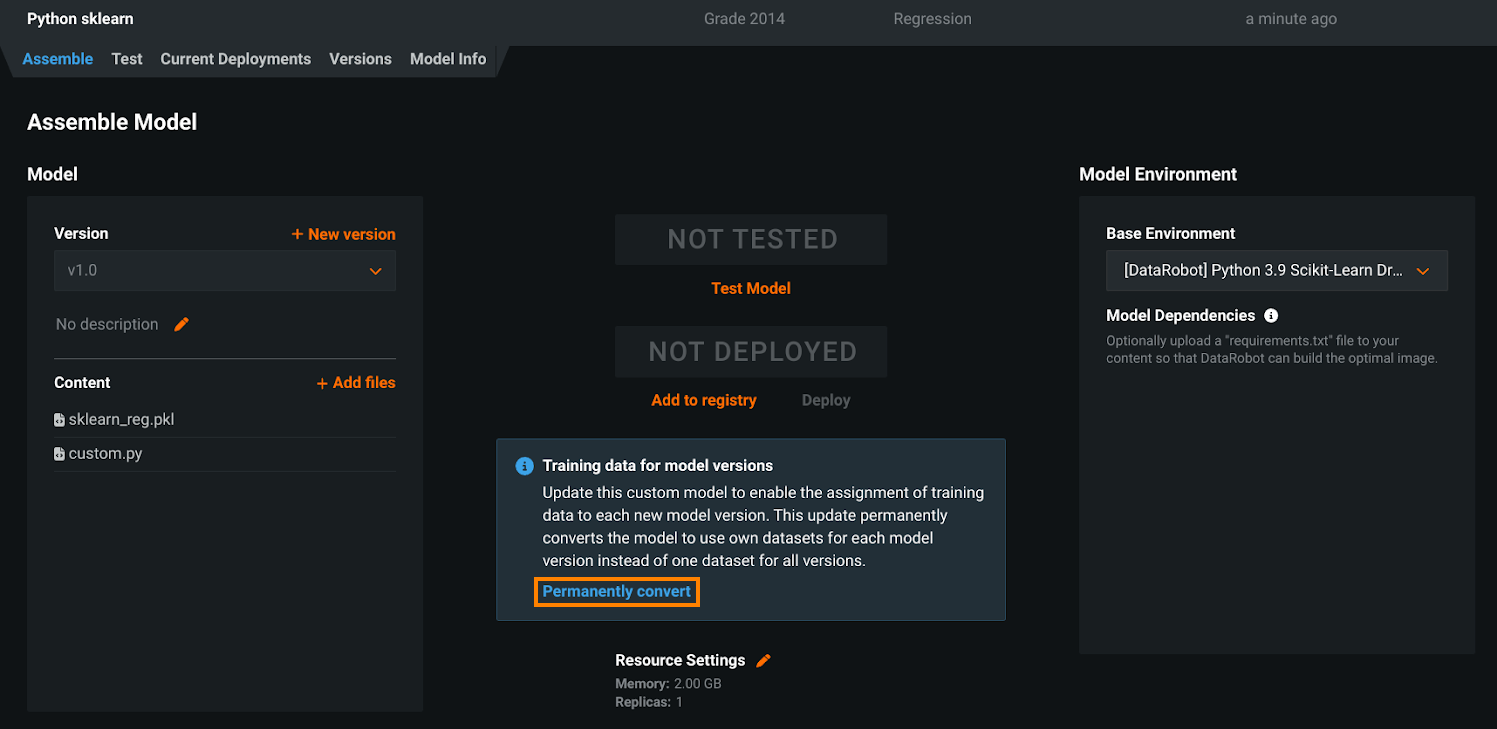
The automatic conversion of any remaining custom models using the “per custom model” method will occur automatically when the deprecation period ends, assigning the training data at the custom model version level. For most users, no action is required; however, if you have any remaining automation relying on unconverted custom models using the “per custom model” assignment method, you should update them to support the “per custom model version” method to avoid any gaps in functionality.
For a summary of the assignment method changes, you can view the Custom model training data assignment update documentation.
Tableau extension removal¶
DataRobot previously offered two Tableau Extensions, Insights, and What-If, that have now been deprecated and removed from the application. The extensions have also been removed from the Tableau store.
All product and company names are trademarks™ or registered® trademarks of their respective holders. Use of them does not imply any affiliation with or endorsement by them.